The impact of technology on different areas of business is unstoppable. The ML implementation solidly changed the game rules. It is also making companies more agile. Machine Learning is helping e-commerce businesses generate revenue in ways they never could previously. There are a number of ways in which we can unleash the full potential of an e-commerce business. And one of them is price optimization.
The overall digitization of the market in recent years no longer allows the use of traditional methods of price optimization for companies that want to stay afloat. Traditional price optimization includes regularly checking and monitoring (e.g. with AB testing) to make sure that prices are aligned with current market conditions, and that they are performing to an optimal degree. This process costs companies a lot of time and effort to manage effectively. Even worse, many companies do not have a good monitoring process in place. Machine learning technology is proving to be a major component in the realm of price optimization, as it is able to address many of the challenges that retailers currently face.
Pricing Strategies
The pricing strategy is an indispensable part of businesses. The balance between margin, price, and selling level is essential and affects all other systems. Therefore, designing a proper pricing plan that ensures business success is critical. It forms the key variable in the financial modeling and affects its income, profits, and investments in the long term.
You must first determine your business goals based on the individual key factors, which each company chooses herself according to the planned result. That is the critical first step of the pricing strategy puzzle. It also involves changing and adjusting the price of goods and services in response to market factors. Corporate image, geographic locations, the ability to provide discounts, and assessing the consumer base’s price sensitivity are usually the most common circumstances of influence. The next step includes market research and evaluation of the level of competition relative to consumer preferences.
Some of the most common types of pricing strategies:
Cost-plus Pricing – the most time-saving way. This strategy combines all the contributing costs for the unit to be sold, adding a fixed percentage as a margin. This strategy is widely used since it’s easy to justify and is typically fair and non-discriminatory. The major drawback of cost-plus pricing is that the customer is not considered.
Psychological Pricing – for increased demand by creating an illusion of enhanced value for the consumer. This strategy relies on the customer favoring one wording over another even though they’re the exact same deal. Setting the price of a watch at $199 is likely to attract more new customers than setting it at $200, even though the actual price difference is quite small.
Premium Pricing – businesses set costs higher because they have a unique product or brand that no one can compete with. You can use this strategy if you have a considerable competitive advantage and know that you can charge a higher price without being undercut by a product of similar quality. To perceive products as worth the higher price tag, a business has to work hard in all directions like the product’s packaging, the store’s decor, and the marketing strategy to create the perception of value.
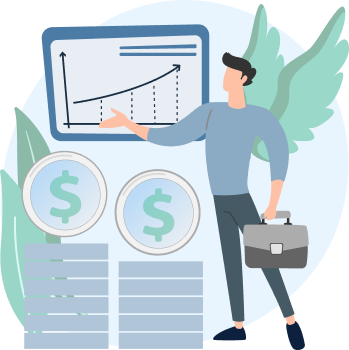
Price Optimization and Automation
They caused a real revolution in the field of pricing optimization and pricing strategy. such technologies have helped to analyze large volumes of data and identify certain regularities there. Machine learning and Artificial Intelligence have automated many pricing methods, making them more practical, fast, and affordable. Here are those of them that are the most effective and popular:
Dynamic Pricing
It involves adjusting the price online, based on changes in demand, supply, or other market conditions. According to AI and ML technologies, the analysis of large volumes of data has become available.
Predictive Analytics
Future results are formed owing to the symbiosis of Statistical Methods and Machine Learning methods. They analyze historical data. Business owners use predictive analytics to optimize pricing by looking at customer behavior and other factors that affect sales.
Demand Forecasting
Pop demand forecasting is built on the basis of historical data, market trends, and many other factors that can affect sales. AI and ML methods can analyze a large amount of data and automate the process of identifying patterns. It helps predict potential demand and uses it to optimize strategies and prices.
Companies using Machine Learning and Artificial Intelligence in the field of price optimization are market leaders. Thanks to the automation of routine processes, they achieve better and more accurate results. This allows them to attract more customers and strengthen their position in the market.
Why Is It Worth Implementing?
However, manual price management remains the most popular method. If a business only has a handful of products and customers, this method can be worthwhile. But when we talk about tens of thousands of SKUs and thousands of customers, this method becomes increasingly complicated and partial to error. In reality, manual efforts to do optimization well in this large field are not justified – which is where machine learning-based price optimization comes in.
Price optimization allows businesses to make informed decisions based on real customer and market data. Usually, the analysis process takes into account a lot of factors and so all your actions will be as accurate as possible, and not guesses. That helps to maximize sales or profitability in accordance.
Dynamic Pricing Optimization
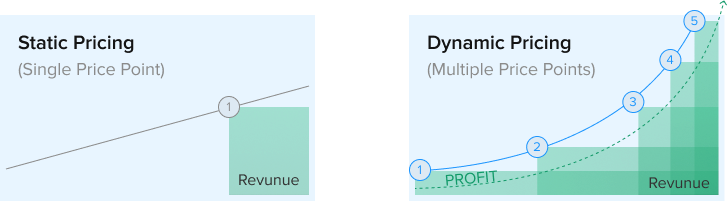
A good rule of thumb to remember:
“Your customer base won’t purchase your product if you price it too high, but your business won’t be able to cover expenses if you price it too low.”
Dynamic pricing is the concept of selling the same product at different prices based on the changing dynamics of the current market demand. This pricing strategy is flexible and variable prices on its products and services depending on any number of standalone or competing factors. The decision-making process behind the dynamic pricing model is quite impressive.
How Does This Work?
To use dynamic pricing algorithms, you need to understand how they work. They evaluate how the price changes from the demand. You can do it by following these steps:
- Calculation of historical data, which would take into account prices, price points, sales, and market demand;
- Calculation of circumstances or elements that affect the price;
- Development of a mathematical model that will work on the basis of significant parameters;
- Running a model that uses new data.
“Machine-learning robots” are working for you to develop new algorithmic models based on the market demands and your competitors’ actions. This data-driven process allows companies to adjust the prices of their goods continuously within seconds without losing a possible profit. It helps to improve their sales conversion rates and ascertain optimal pricing that can balance out profit margins and conversion rates.
There are many known retailers who are already harnessing the power of dynamic pricing. It definitely works better than other strategies and allows you to manage your processes more effectively and rationally. These include well-known international brands which use machine learning-based pricing cases:
- Zara as a fashion company determines its entry-level prices via AI, and lets prices react to trends in an automated way. Following this way, Zara has to sell only 15 to 20 percent of its products at discounted prices, as opposed to 30 to 40 percent at other European retailers.
- Ralph Lauren is using machine learning to sell fewer garments via markdown prices and manage inventory more efficiently.
- Boohoo, and Shein as preachers of fast fashion are known to use machine learning to achieve their business goals despite low entry prices.
Predictive Analytics for Price Optimisation
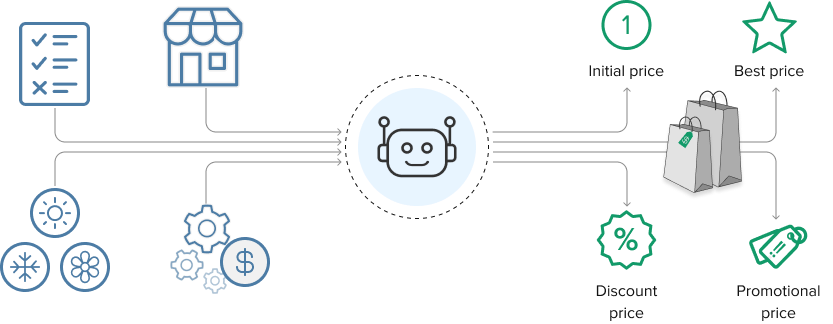
We already know what predictive analytics is. Now should we figure out how it works?
- To begin with, it is necessary to collect analytical data, on the basis of which the final assessment of pricing will be calculated. This can be influenced by various factors, from customer behavior to market trends.
- After collecting data, it must be cleaned to avoid inconsistencies, errors, or fakes. All this can affect the accuracy of the analysis.
- Next, all data must be converted into a format, which will be used for further analysis. This process involves selecting the appropriate variables and converting them into the format to be used.
- At the end of these processes, a model is selected that meets the set requirements. These may include a regression model, a classification model, or a time series model. After that, it is trained on the basis of a decision tree, neural networks, or regression analysis.
- After completing the training of the model, it is checked. This can be understood as a comparison of the actual values with the predicted ones. This is how discrepancies are revealed and the model is improved.
- If all stages are successful, the model is deployed in a production environment, where it predicts future results and optimization of the starting pricing.
Many companies use this price optimization technology to achieve better results in the market. For example, Amazon adjusts prices based on demand, competition, and inventory. In its criteria, Uber also includes traffic conditions and road factors that can affect the price of its services. Airbnb determines the optimal price for listings by analyzing demand, location, or seasonality. With Predictive Analytics in Price Optimization, General Motors sets vehicle prices based on market demand, competition, or vehicle characteristics.
Demand Forecasting for Price Optimisation
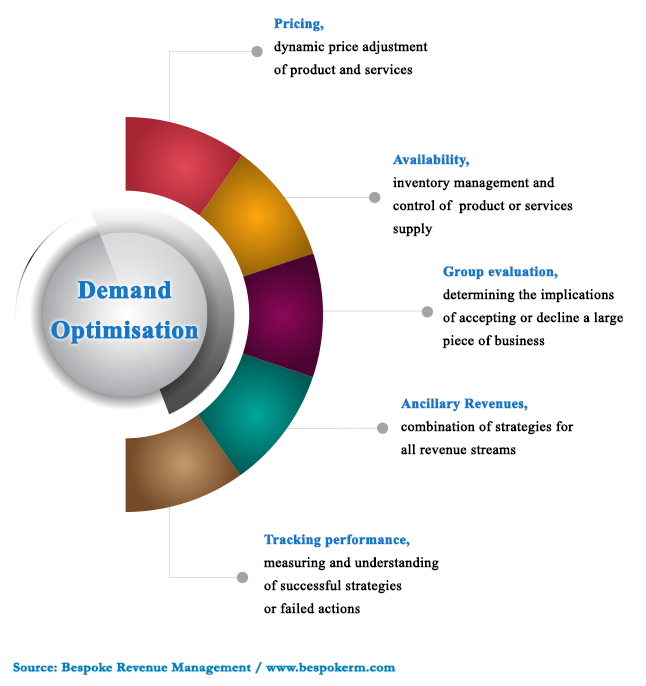
Demand forecasting is also an important price optimization technique. Businesses can use it for pricing and forecasting changes in consumer demand and income.
Demand forecasting: How to?
- Step 1. Collect data that is important in researching the demand for your business or service.
- Step 2. Data analysis. Both time series analysis and regression analysis can be used here. It will show patterns and trends.
- Step 3. Model selection. According to your analysis, you can choose a Moving Average Model, ARIMA, or others.
- Step 4. Forecast. You can use the model to both predict future demand and estimate how prices might affect demand and revenue.
- Step 5. Price optimization. Based on their previous steps, business owners can develop pricing systems that will attract customers’ attention and increase their demand. This can be about the pricing of certain products or even the strategy itself, through A/B testing.
- Step 6. Observation. After you implement changes to pricing, you need to monitor whether your strategy is delivering the expected profit and whether it needs to be refined.
Successful companies have long used demand forecasting to increase customer loyalty and optimize revenue.
- Booking.com uses technology to set optimal prices for its hotels and vacation rentals.
- Delta Air Lines analyzes seasonal and market trends to achieve comfortable pricing for its services.
- Coca-Cola is also among the companies that use demand forecasting. With the help of Artificial Intelligence and Machine Learning algorithms, the company analyzes consumer trends, consumer sentiment, competitors, and other factors. This helps them optimize their prices and make them convenient for their customers.
Benefits of Machine Learning for Price Optimisation
Machine learning pricing optimization that is based on Python gives the advantage to do all processes automatically. You spend time only to create a retail price optimization project with appropriate settings that take into account the influencing factors that are important for your business pricing strategy. For example, you can evaluate data like historical sales and transaction data, seasonal changes, weather conditions, inventory levels, product features, marketing campaigns, and others.
Price optimization using Machine Learning to analyze all of the variables and determine the possible effects of price changes on sales. Algorithms can also deliver real-time insights to help you make your other operations more efficient.
So, you can get:
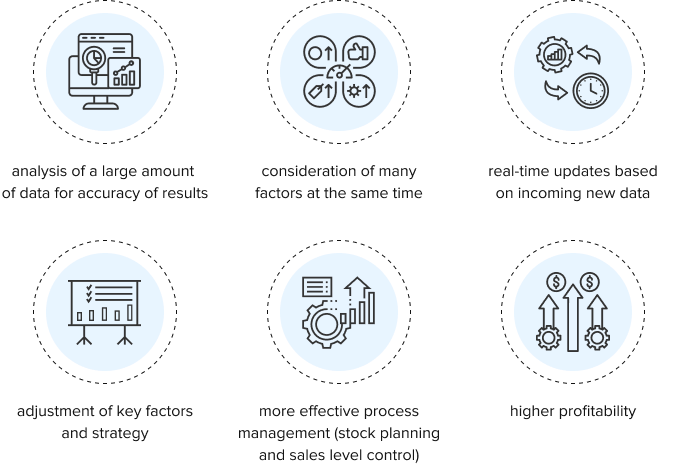
Conclusion
Machine learning algorithms for e-commerce are a major component that allows us to lose many of the challenges that retailers currently face. This gives an opportunity to analyze much larger data sets and take into account significantly more variables than is possible with traditional pricing. Therefore, retailers can adjust the model relative to their own sales targets. That will more precisely determine the acceptable price and gives the advantage to create predictions of how demand will fluctuate as a result of changes in conditions.
Don’t delay and implement new technologies and optimization algorithms for your business today. You can book a consultation with our specialists who will choose the best solutions for you.