Intelligent Generation of Interior Designs
Technologies:
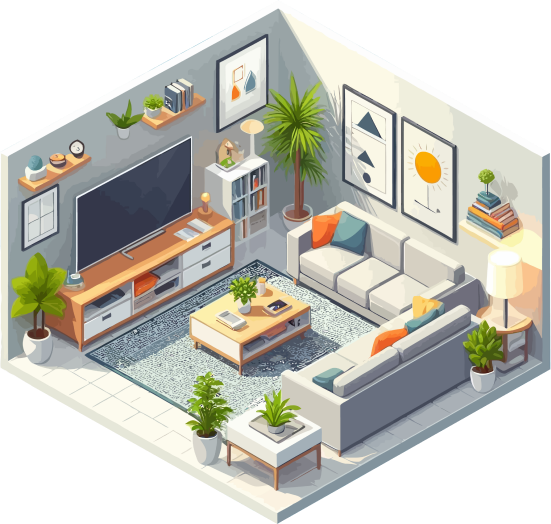
About the Client
Our client was a foreign technology company developing mobile applications using Artificial Intelligence. They create advanced and revolutionary applications that help end customers solve many tasks in various areas, from photography to utility payments.
Business Context
The client wanted to create an application for interior design. Its essence was a convenient, fast, and easy-to-use application for visualizing changes in the existing interior of the room using a photo. That is, the end user, after taking a picture of the space that he would like to change, could set certain parameters for the changes, and receive a reformatted image with an updated design and solution.
Our Data Scientists joined the client’s team to:
- Write a prompt for the model to get the expected design and room type.
- Write a script to create communication between the user and the model.
Amazinum Data Scientists In Action
The client provided Amazinum’s Data Scientists with data on the types of rooms (living room, bedroom, bathroom, kitchen, dining room, home office), a partially written negative prompt template, and 9 styles (tropical, zen, mid-century, minimalistic, modern, farm house, industrial, rustik, vintage) the application should accept and support.
First of all, our specialists started looking for a model that would regenerate pictures, with the condition that the selected categories would remain unchanged. Thus, the choice fell on the Inpainting models (DreamShaper XL). fal.ai was used to wrap the model and setup all parameters.
After that, the Data Scientists started working on the dataset, which consisted of two parts. For the first, it was necessary to collect data on interiors that can be redesigned, for the second – interior styles specified by the client. To correctly validate the choice of the model, specialists looked for references with which the practicality and functionality of the interior could be compared.
After the data set, Amazinum Data Scientists began work on the project. It was necessary to give the model the clearest explanation of what you should get at the exit. Thus, we described in detail each of the 9 given interior styles. Based on this information, specialists created two prompts – negative and positive. The positive remembered what changes it should make, the negative – which categories should remain unchanged. After that, testing was carried out based on certain images.
For everything to work harmoniously, it was necessary to set up communication that would combine the human-model-human links. To do this, our data scientists wrote a Python script, which was wrapped in pyodide, to form an interaction between the user and the model, extract images from the queue, and send them back to the user. Pyodide was used to integrate the Python script into the node.js script without additional effort.
Challenges for the Amazinum Team
Among the difficulties faced by our specialists were:
- Short deadlines for completing the task
- Absence of expert assessment or reference answer
- Lack of clear validation of the interior design at the exit.