Over the years, AI and oceanography have created a powerful synthesis that can shed light on many important issues related to protecting and exploring the earth’s water surfaces. The emerging challenges require more efficient, accurate, and scalable solutions for marine ecology.
Machine learning and AI offer new insights through which we can explore the oceans, reduce water pollution, monitor marine biodiversity, or predict tides. All of this remains promising and offers many new and effective solutions.
According to the study, ocean industries such as fishing, shipping, and energy generation generate at least $1.5 trillion in economic activity each year and support 31 million jobs.
Problems faced by traditional methods
Traditional methods of researching the components of the ocean are gradually exhausting themselves, due to which they currently face a considerable number of problems, such as:
The Danger of the Sea Depths For the Human Body
Oceanographers and marine biologists regularly face many problems, most of which are related to the danger to the human body. The deep oceans are inaccessible, devoid of light, and subject to extreme pressure, directly affecting research and making it risky and resource-intensive. The tasks faced by traditional methods require huge resources and time, and they are dangerous for people. Also, many changes and phenomena are invisible to the human eye. According to the National Oceanic and Atmospheric Administration (NOAA), this capability is key because more than 80% of Earth’s oceans remain uncharted, uncharted, and unexplored
A Large Amount of Data
Artificial Intelligence becomes crucial in processing a large amount of data, recognizing patterns, or tasks that require large resources. Data captured by tag-and-track devices, underwater cameras, and remote sensing technologies can be easily analyzed using Artificial Intelligence. This answers many questions such as migration patterns, predator-prey relationships and responses to environmental stressors.
“A big ocean needs big data. Researchers are collecting large quantities of visual data to observe life in the ocean. How can we possibly process all this information without automation? Machine learning provides an exciting pathway forward – MBARI Principal Engineer Kakani Katija
The Scale of the Oceans and the Variability of Their Ecosystems
The scale of the world’s oceans, and their variability, require a complex interpretation of complex biological, chemical, and physical interactions. Because of this, with traditional processing, the data is often incomplete and ambiguous.
Spatial and Temporal Resolution of the Data
Traditional methods such as diver surveys and boat sampling require considerable costs, time and resources but are still limited to small local areas. Therefore, a comprehensive understanding of marine ecosystems requires large-scale models that can be provided by Artificial Intelligence. In addition, the rapid pace of changes in the environment can make it difficult to interpret data manually.
How can AI help tackle plastic in the ocean and its Impact on Marine Ecology
Plastic waste in the ocean is an issue that is becoming more and more acute every year. Negative effects on the reproductive system of animals and fish, the destruction of ocean fauna, the disappearance of rivers due to pollution, the emission of chemicals that penetrate every particle of our planet – this is far from a complete list, this is only its beginning.
The world produces more than 430 million tons of plastic per year. Two-thirds become waste after the first use. According to the Ocean Conservatory, eleven million metric tons of plastic enter the ocean each year, on top of the approximately 200 million metric tons already flowing through our marine environment.
Nicola Simpson, head of the United Nations Development Programme’s Barbados and Eastern Caribbean Blue Economy Promotion Lab, says that at these production rates, there will be more plastic than fish in the ocean by mid-century.
Therefore, this is the #1 question facing our generation. We need to take all the necessary measures and direct all efforts to save the ocean, correct our mistakes and leave to our descendants the unique nature that we have been given.
In this matter, the technologies of Artificial Intelligence and Machine Learning have considerable potential. And now, we’ll tell you how it can help tackle the problem of ocean plastic pollution.
Automatic Analysis Using Satellite Images
Researchers from Wageningen University and EPFL published a study in Cell iScience in 2023 in which they developed an AI-based detector that estimates the probability of marine debris shown in satellite images. This technology can help get rid of plastic waste by removing it with the help of ships. The European Space Agency’s Sentinel-2 satellite records the accumulation of waste in the seaThe images are freely available and require analysis by Artificial Intelligence and deep neural networks, as they contain terabytes of data.
The founders of the study are Davis Tuya, associate professor at EPFL and director of the Computational Science for Environmental and Earth Observation (ECEO) Laboratory in Zion, Mark Rooswurm, associate professor at Wageningen University and former researcher at EPFL, and Sushen Jilla Venkatesa Environmental Computational Science and Earth Observation (ECEO) Laboratory.logy
Mark Rooswurm says about this technology,“These models are trained on examples provided by oceanographers and remote sensing specialists who have visually identified several thousand marine debris in satellite images around the world. In this way, they “taught” the model to recognize plastic waste.
How it works ?
Every 2-5 days, Sentinel-2 captures images of coastal areas around the world. The detector, based on Artificial Intelligence, evaluates each pixel in satellite images to detect debris. It learns according to the principles of artificial intelligence. In this way, it works on data to make the best use of limited training data. The detector uses Computer Vision algorithms to recognize objects visible on the screen and provide this data to oceanographers and remote sensing experts.
Mark Rooswurm, associate professor at Wageningen University and former EPFL researcher, adds: “The detector remains accurate even in more challenging conditions. For example, when cloud cover and atmospheric haze make it difficult for existing models to accurately identify marine debris.”
The detection model can also spot debris in daily PlanetScope images taken from cubic nanosatellites.
“Combining weekly Sentinel-2 with daily PlanetScope collections can close the gap in continuous daily monitoring,” Russwurm says. “In addition, PlanetScope and Sentinel-2 sometimes record the same area of marine debris on the same day within minutes of each other. This double image of the same object in two locations shows the direction of drift due to wind and oceanic thin studies.”
Research in these areas will be continued by Mark Rooswurm from the University of Wageningen, together with Professor Tim van Emmerik, an expert on river plastics, and partners in the Netherlands, such as Ocean Cleanup. The company collects plastic in the open ocean on special ships. This is a collaboration for the AI for Detection of Plastics with Tracking (ADOPT) joint project in collaboration with the Swiss Data Science Center (a joint venture between ETH Zurich and EPFL) and EPFL Prof. Davies Tuia, Dr. Emmanuel Dalsasso and Prof. Marc. Rooswurm.
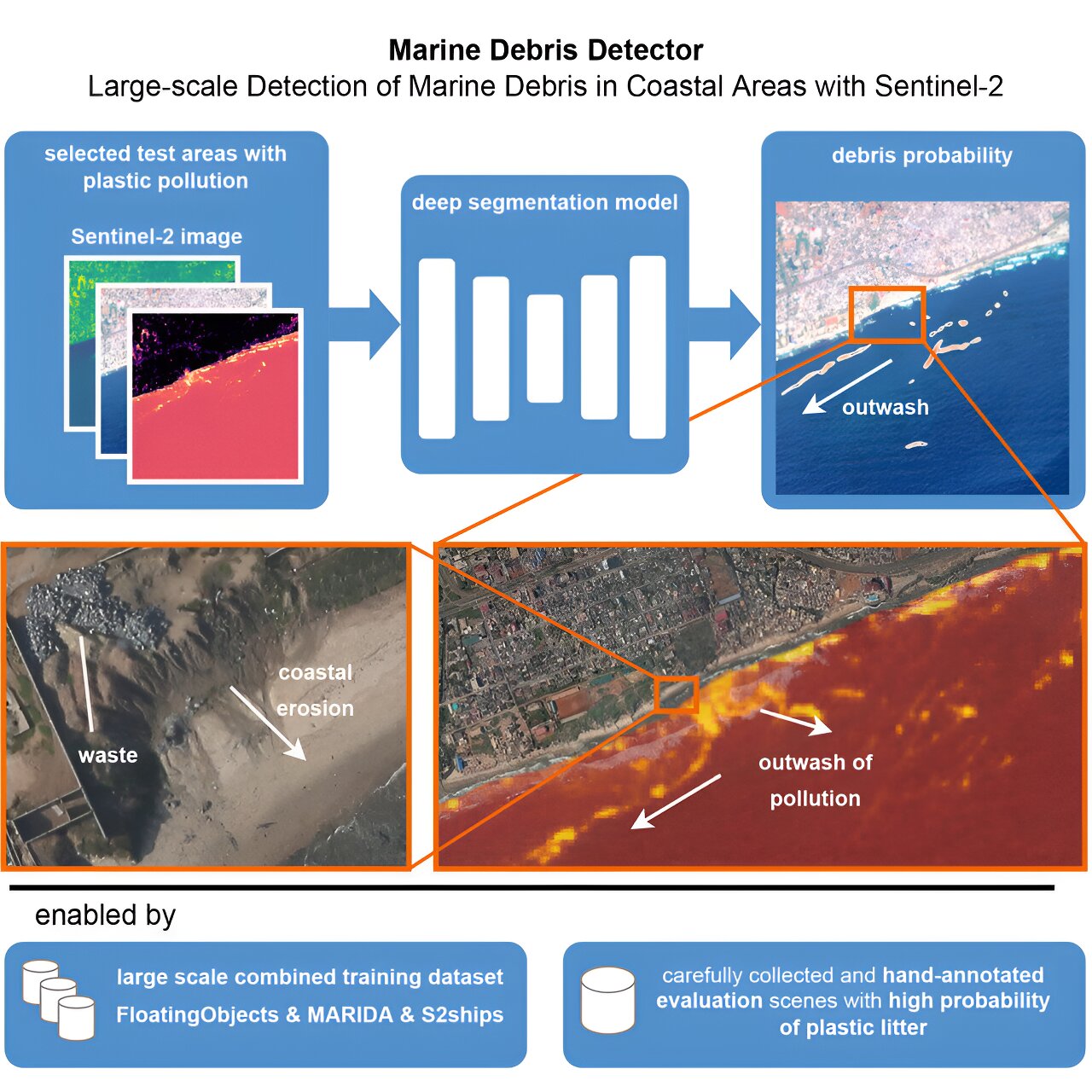
Source: Link
The Ocean Cleanup
In 2021, the NGO The Ocean Cleanup offered its own AI-powered monitoring and mapping tool.
Using Machine Learning technologies, Ocean Cleanup detects plastic pollution and models its movement in the ocean. This helps passive cleaning systems remove plastic.
The model was trained on a huge number of source images. A neural network detects objects on them. It consists of a series of mathematical equations with different settings called weights. Thanks to this, the neural network learned to detect the object based on the training examples it received by passing the example images.
The training images also needed a lot of work. To obtain them, Ocean Cleanup tagged approximately 4,000 examples of objects in photographs from their Aerial Expedition (2016) mission and the 2018 System 00.1 (“Wilson”) voyage. The transformed images were fed to the model for training, after which a dataset of 18,589 images was usable.
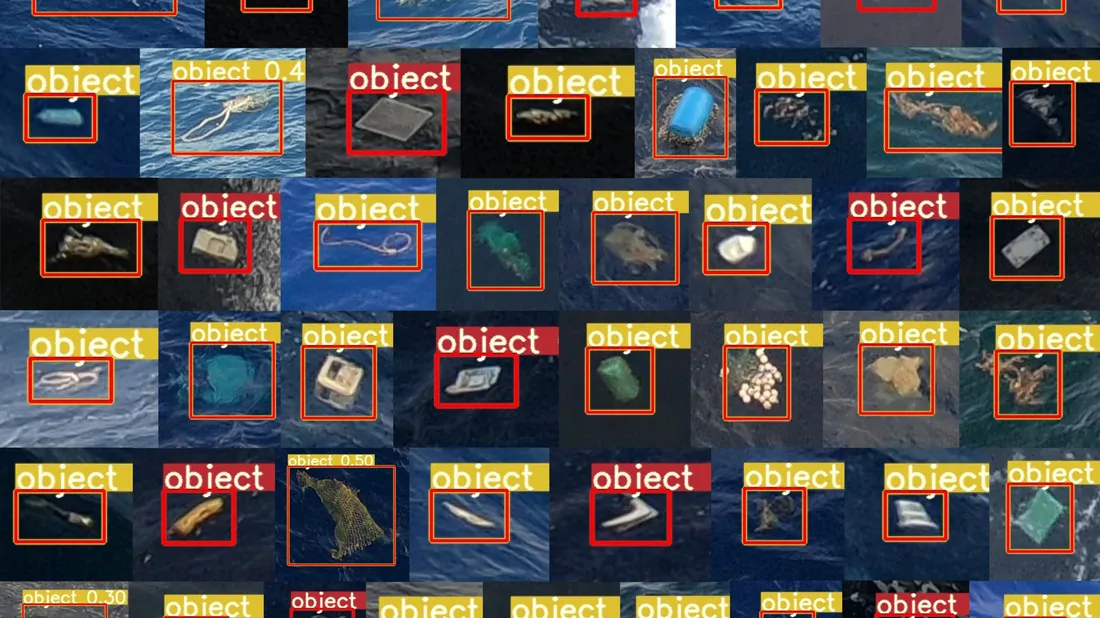
Source: Link
In addition, the Ocean Cleanup team collected a dataset from aboard the Maersk Transporter during their System 001/B mission in 2019. In doing so, they captured more than 100,000 time-lapse photos from the port and starboard sides of the vessel. The photos were geotagged, which helped to obtain unique GPS coordinates.
Knowing the location of each object, the Ocean Cleanup team grouped the GPS coordinates of the photos into different sectors. From this, they obtained an estimated number density per transect by grouping the detected objects in the transect and dividing this by its total area. In this way, it allows the creation of a map of the concentration of plastic in the ocean.
By 2024, Ocean Cleanup plans to reduce ocean plastic by 90% by combining the two systems and scaling them up.
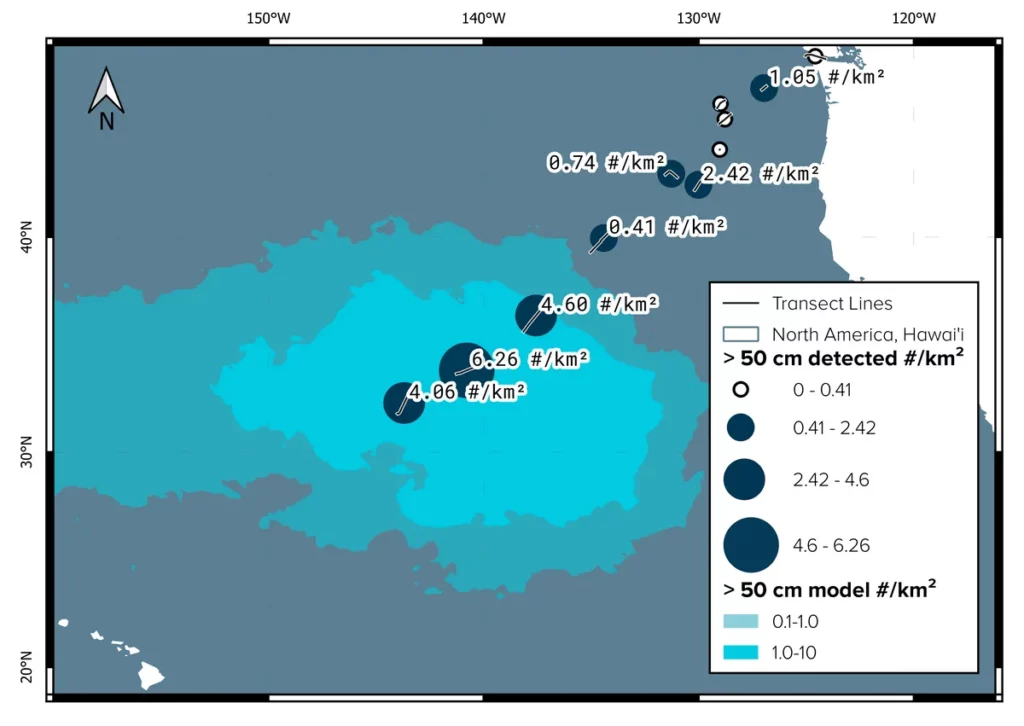
Source: Link
Razer and Clearbot
In 2021 Razer, in partnership with ClearBot, created an automated robot that works based on Machine Learning and Artificial Intelligence and detects marine plastic at a distance of two meters in turbulent water. The robot runs on solar energy and can collect up to 250 kg of plastic in just one cycle.
ClearBot’s CEO says, “The Razer team’s action-oriented approach to tackling marine litter has been extremely impressive. We are grateful to the team that volunteered their time for this project. With the new model, we are confident that we will be able to expand our global reach to protect marine waters, starting with partners including seaport operators in Asia and non-governmental organizations who have already expressed interest. Together with Razer, we look forward to making positive changes in the world.”
That year, for World Oceans Day, ClearBot called for pictures of marine plastic debris to be uploaded to its website. This is usually found in open water. AI models were trained to detect waste based on these photos.
Gringgo Indonesia Foundation
For Indonesia, the problem of plastic waste is very acute. Much of the trash that is located along the coastline can end up in the ocean. That is why the Gringgo Indonesia Foundation decided to solve this problem with the help of technology and also with the support of Google.
In 2019, Gringgo was named one of 20 grantees of the Google AI Impact Challenge, giving them resources to get started.
Gringgo Indonesia Foundation aimed to create an image recognition tool that could classify materials and give them a monetary value. This would help reduce ocean pollution, improve public awareness, and help create an economic model for waste management.
In 2017, they launched several apps that allowed recycling workers to track the amount and type of waste they collect. This saved time by offering a more organized route and profit estimation. Using Image Recognition technology, waste management workers can take pictures of trash and identify its value.
Thanks to this, employees of waste processing services can estimate the market value of materials and optimize their activities.
Febriadi Pratama, CTO & co-founder at Gringgo says: “Within a year of launching the apps, we were able to increase recycling rates by 35 percent in our first pilot village, Sanur Kaja in Bali. We also launched a public app that connects people with services collection of waste in their homes”.
With the support of Google, the Gringgo Indonesia Foundation is working with the Indonesian startup Datanest. Together, they are working to create an image recognition tool using Google’s TensorFlow machine learning platform. The goal of the project is to enable waste workers to better analyze and classify waste, as well as quantify its value.
Open Ocean Engineering
Hong Kong startup Open Ocean Engineering aims to fight pollution with zero-emission automated boats called Clearbots. They can scoop trash out of the water and bring it ashore for recycling.
In 2022, Open Ocean Engineering won the Alibaba Group Jumpstarter 2022 Global Pitch Competition for startups.
Clearbot Neo is an automated boat that sails through harbors, canals or rivers to collect trash that would otherwise end up in the ocean. Siddhant Gupta and Utkarsh Goel, the creators of Clearbot, were inspired to create it after a trip to Bali. There, local workers removed garbage from the sea in small boats. That is why they decided to automate this process, and in conclusion, Clearbot was born.
The robot works from an electric motor on solar batteries. It picks up the debris in the water and collects it. One such robot can collect a metric ton of garbage per day. It can also clean up localized oil or fuel spills if equipped with an individual boom. In this case, it can collect up to 15 liters of pollutants per day. Clearbot Neo has a dual-camera detection system. This allows it to monitor the surface of the water look for debris or any obstructions and scoop the debris onto an onboard conveyor between the twin hulls. At the same time, another camera photographs the garbage that is already entering the conveyor. The image and location are received by the corresponding system hosted on the Microsoft Azure platform.
In the spring of 2020, Open Ocean Engineering received an AI for Earth grant from Microsoft. This helped them create their model, based on Artificial Intelligence on the Azure platform.
“We’re figuring out how the trash gets into the water in the first place,” Gupta said. “This adds significant transparency to the sea cleanup process. We’re collecting data about what’s in the water, what’s the composition of the things that are in there, how much of it can be recycled, and what materials we should focus on.”
The Potential of AI in the Study and Understanding of the Marine Ecosystem and Impact AI on Marine Ecology
Artificial Intelligence has already made its contribution to oceanography and the preservation of the natural environment of water resources.
Impact of AI on Understanding of Marine Fauna
Understanding the behavior of marine species is an important aspect of underwater resources research. However, many factors influence the movement patterns or behavior of creatures. Among them, for example, the availability of food, habitat conditions, human influence or climate change. That is why researchers are increasingly using Artificial Intelligence and advanced technologies to predict and interpret the behavior of marine species. This allows you to achieve a level and scale of accuracy that you could only dream of before. With AI’s ability to analyze large data sets, scientists can open new frontiers in species monitoring and management.
Plankton and Fish Stocks Monitoring
With the help of Artificial Intelligence, marine biologists can better monitor plankton and fish stocks, using technology to count fish, monitor their size and even distinguish one type of fish from another. Artificial Intelligence has automated many tasks that required a lot of time, resources and attention. In addition, AI can distinguish between individual fish species, helping researchers to study and understand the lifespan and behavior of a particular species in detail.
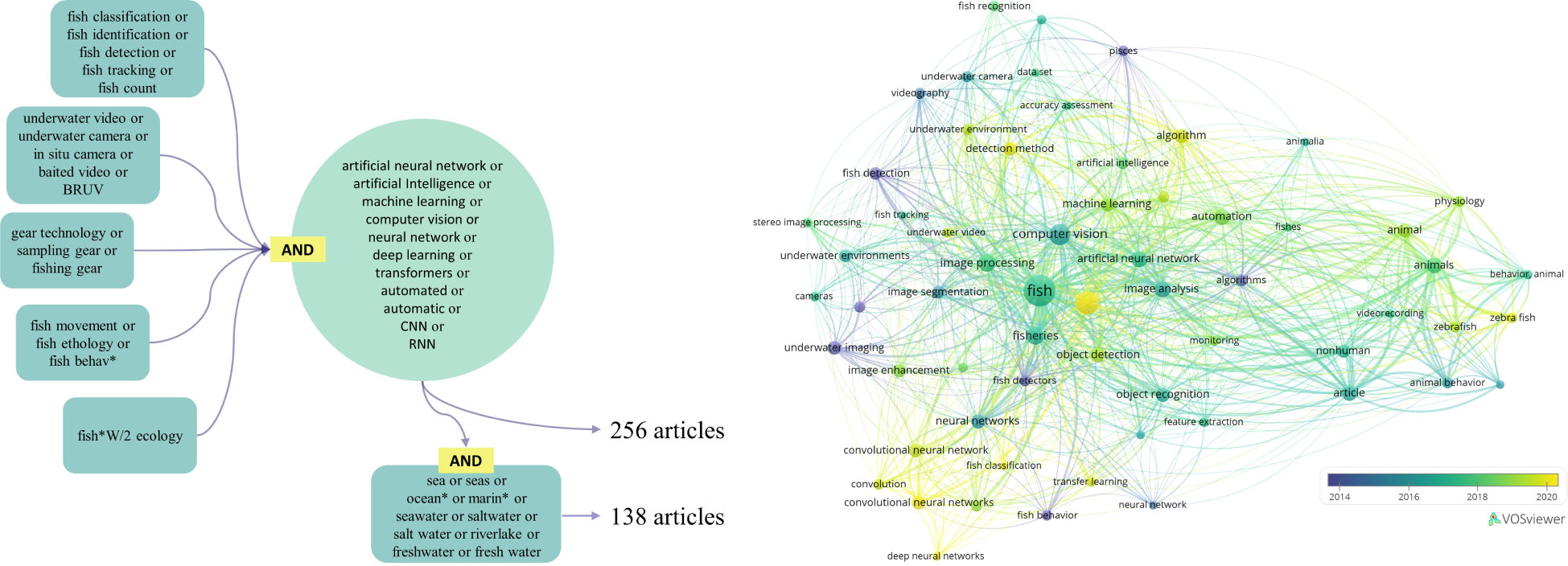
Understanding the Seal Population
Deep learning models help ecologists and researchers count seals, based on archival photos, and monitor their populations. Now, they can sort 100 images in less than one minute! Imagine how long it would take a human to complete the same task. Deep Learning algorithms eliminate the need for pre-imagining individual seals and counting them on each image. Now, researchers can understand the evolution of the seal population much faster, much more accurately and much more fun!
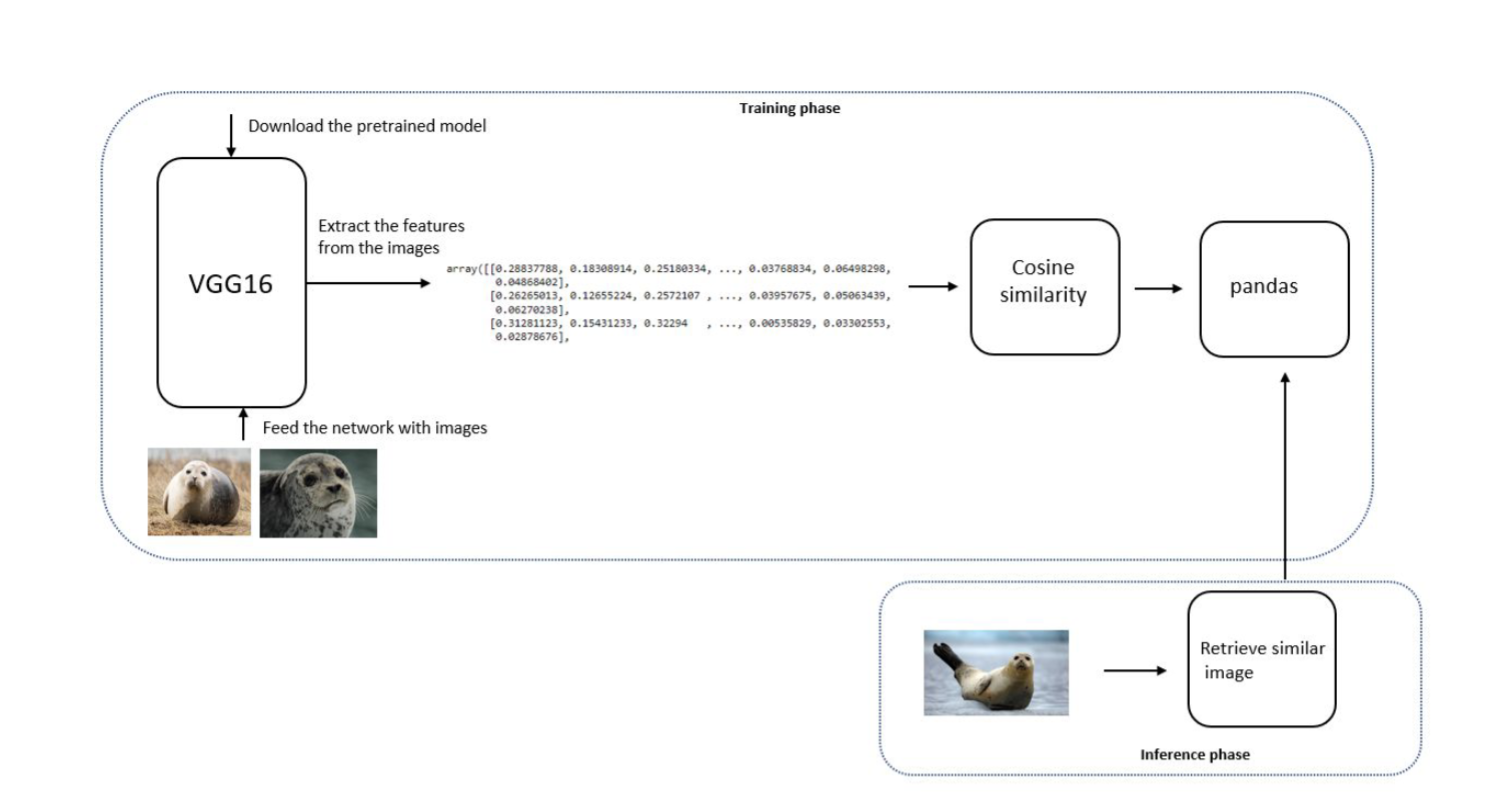
Recognition of behavioral patterns
Machine learning algorithms can detect and recognize specific patterns of behavior that indicate routine activities or reactions to environmental stimuli. This is possible based on visual and acoustic data. AI can also track behaviors such as breeding and nesting based on video. Computer vision can identify and detect individual species. In this way, researchers can study populations, behaviors, or correct anomalies.
For example: By analyzing vocalization patterns captured by underwater microphones (hydrophones), AI can track whales’ mating patterns. This kind of technology can distinguish the calls of individual species of whales or even identify special acoustic features.
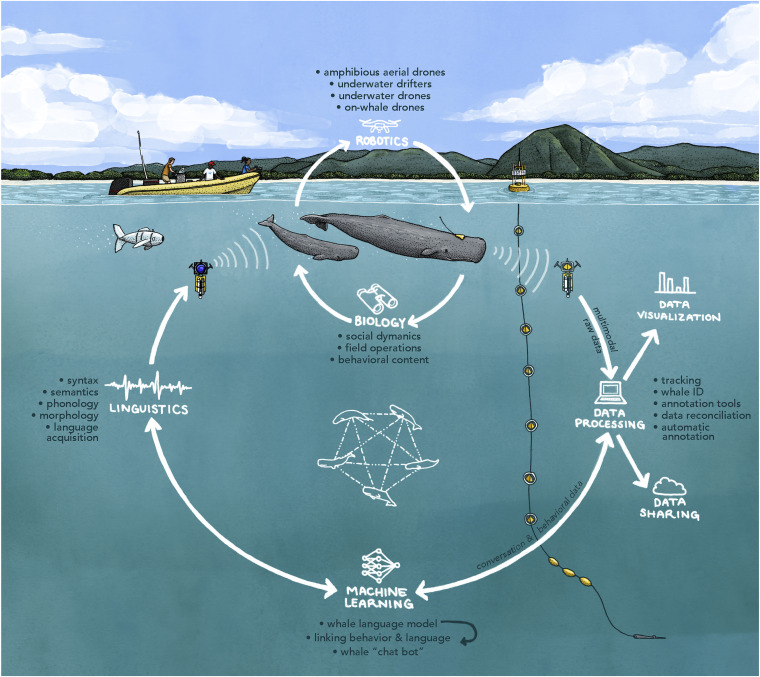
Source: Link
This kind of knowledge is extremely important, scientists are putting a lot of effort into preserving endangered species. We need to do everything possible to understand their behavior or anomalies, to protect their livelihood successfully, and to follow their suggestions.
AI and Maritime Migration Tracking
The study of the migration of marine species with the help of Artificial Intelligence caused a revolution in this field. Satellite tags collect data on animal location, water depth, and temperature. After that, they go to Artificial Intelligence. It processes data on migration routes and patterns and sends them to ecologists. Machine Learning algorithms predict future movements and behaviors based on tracking data. All this gives an understanding of the state of the ocean and helps to respond in time to changes, protect the marine environment, and manage fisheries.
Helping AI in Understanding Eating Behavior
Using sensors on animal tags, such as accelerometers and cameras, AI can observe and interpret the feeding behavior of species in the wild. The health of marine populations directly depends on their feeding behavior and the productivity of their ecosystems. That is why it is important to monitor them and understand the positive or negative factors. Thanks to this, ecologists can receive information about resources, the number of prey in a certain area, or the quality of the habitat. This information will help to respond in time, intervene in the process if necessary, and conduct further research.
For example: during the study of penguin feeding behavior, accelerometers detected shocks that occurred when the penguins grabbed the prey. After that, Artificial Intelligence analyzes the information collected from the devices and provides an overview of the schedule of meals, feeding, duration of meals, etc.
Interactions of marine organisms
Artificial Intelligence also helps to understand the patterns and correlations in the interaction networks of marine inhabitants. Deep Learning algorithms based on genetic, metabolic, and observational data provide insights into the interactions of marine creatures with each other, their interactions with the environment, and environmental changes. Any natural or anthropogenic destruction can affect marine fauna. Therefore, it is important to recognize these changes in time and implement strategies to mitigate the negative consequences.
Example. Artificial Intelligence can help find interdependencies in coral reef ecosystems, which in turn will help determine priority conservation and sustainability measures. Thus, Allen’s coral atlas, using Machine Learning methods, maps and monitors the state of coral reefs by comparing satellite images with field images.
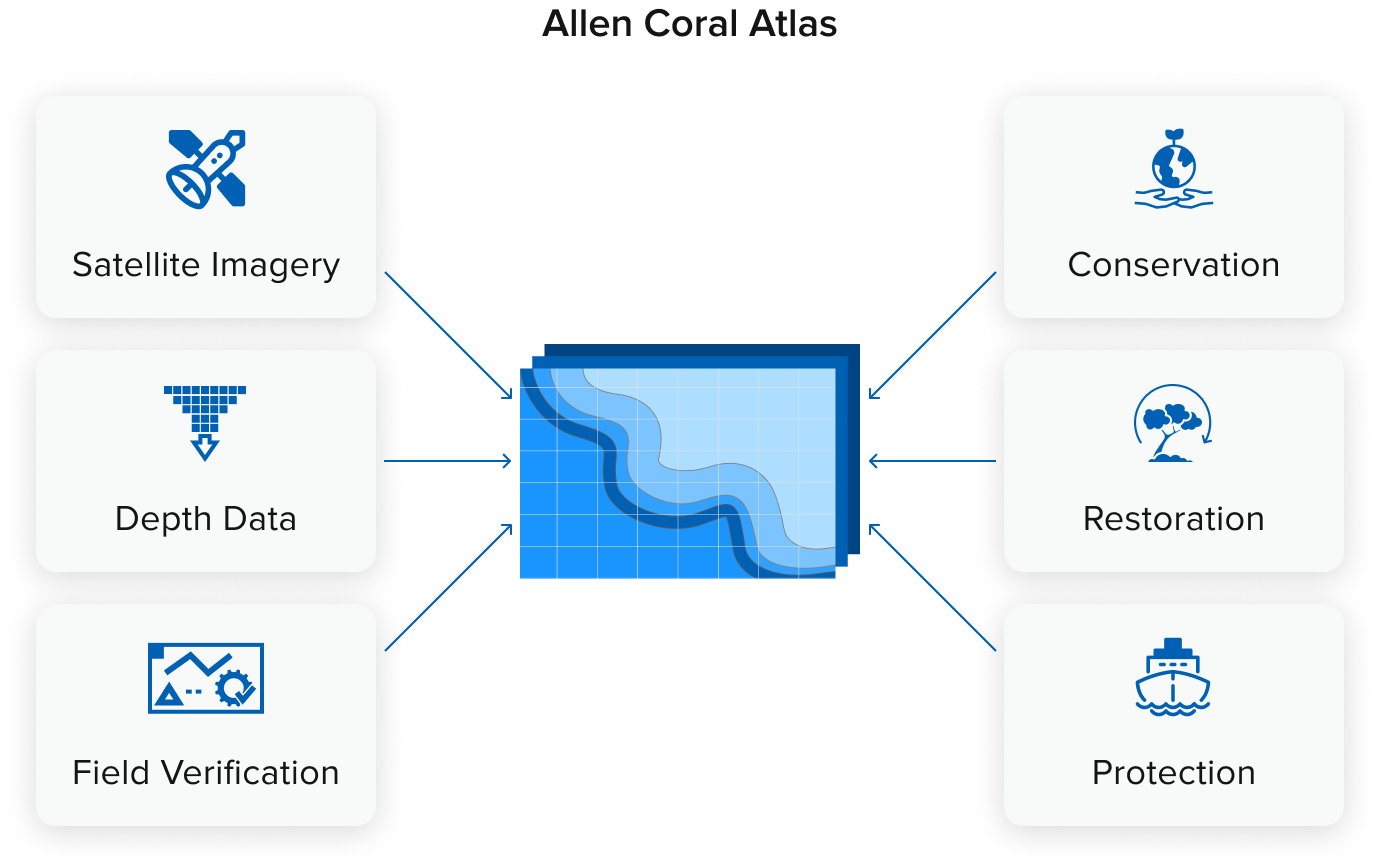
Impact of Artificial Intelligence on the State and Research of the Ocean
Navigation in Unexplored Depths
Underwater drones often face a navigation challenge, as the deep sea has its own dangers and unpredictable terrain. This complicates driving the vehicle and requires occasional human intervention.
Artificial Intelligence algorithms provide drones with the ability to navigate autonomously. They are equipped with sensors such as acoustic doppler, inertial measuring devices and pressure gauges. Thanks to this, drones perceive the environment with high sensitivity. Machine learning integrates sensor data to build real-time maps of the underwater environment. Thanks to the support of machine learning methods, drones adapt to changing conditions and make decisions in a fraction of a second.
This kind of self-guided navigation is critical to the safety and preservation of resources and equipment. Machines controlled by Artificial Intelligence significantly increase the percentage of successful missions, and reduce risks for people, equipment, or ecosystems they cross.
Modeling of Deep-sea Resources
Modeling of seabed resources has reached a new level thanks to collaboration with Machine Learning algorithms. ML technologies have brought an innovative approach to 3D modeling to improve precipitation transport in water or seabed reconstruction.
For example, based on artificial intelligence, scientists created a mathematical model that estimated the distribution of cobalt-rich manganese crust. The model created a 3D color reconstruction of the seabed, based on the analysis of autonomous underwater vehicle (AUV) sensors and a light profile mapping system.
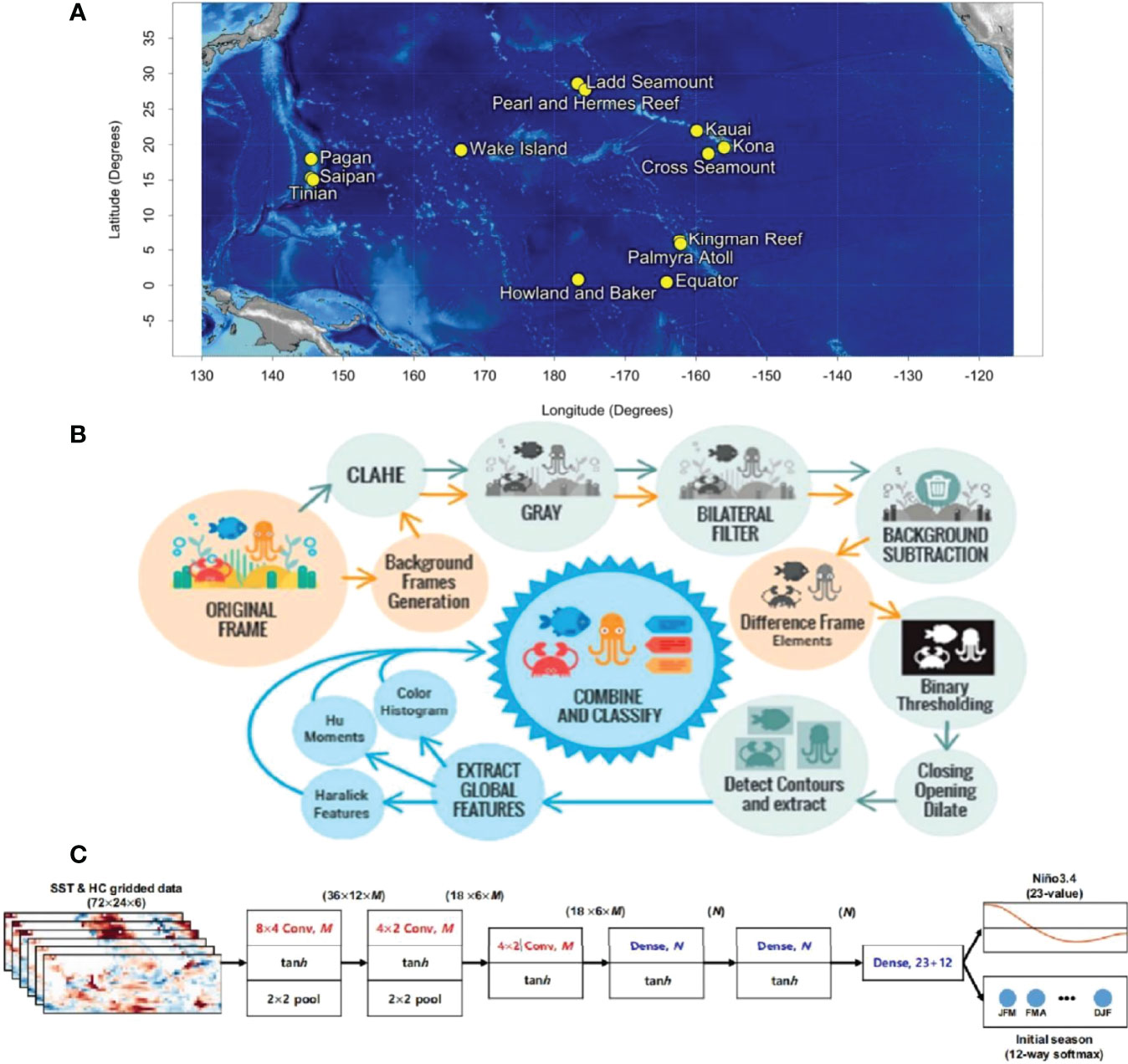
Source: Link
Sensors based on Artificial Intelligence
Special smart sensors inform scientists about the state of the ocean. AI-equipped sensors can detect and assess chemical, biological or physical changes in the ocean ecosystem. Such sensors can be located on buoys, autonomous underwater vehicles (AUV), or marine organisms. This provides comprehensive monitoring of the ocean system and allows timely response to sudden changes.
Because the sensors are equipped with Artificial Intelligence technology, they can continuously record important metrics such as pH level, salinity, temperature, dissolved oxygen, or turbidity. Machine Learning algorithms help sensors adapt and improve, which ultimately increases their predictive capabilities and helps them respond to environmental threats promptly.
Improved Satellite Imagery
Artificial Intelligence can process the vast amount of material it receives from satellite remote sensing to track changes. Machine learning models can recognize chlorophyll concentration, sea ice area, and surface temperature anomalies. They trained on huge datasets of ocean images. Artificial Intelligence processes satellite images and provides comprehensive information about the state of marine ecosystems. Thus, the use of convolutional neural networks helps researchers assess the impact of climate change on the oceans, and identify long-term trends or anomalies.
The Impact of AI On Data Collection
AUVs equipped with Artificial Intelligence technology, such as Computer Vision, can collect vast amounts of visual and acoustic data. Convolutional neural networks of Artificial Intelligence process the collected data. Thanks to this, they can classify biological and geological elements with unprecedented precision. In the previous paragraph, we already wrote that this type of apparatus helps to know the state of the ocean by measuring parameters of temperature, turbidity, pH level or salinity. Such data is amenable to analysis by AI algorithms, and gives scientists a deeper understanding of critical points in the ocean, such as dispersal of chemical plumes, distribution of biological hotspots and thermohaline circulation.
In addition, unmanned aerial vehicles are also equipped with passive acoustic monitoring (PAM) systems. Thanks to this, they can classify the sounds of underwater creatures, analyze geological activity such as underwater earthquakes or landslides. In this case, Artificial Intelligence is included in the process of clustering and analysis of uncontrolled time series. This allows timely detection of seismic processes and monitoring of marine life.
Predictive Models of Artificial Intelligence
Analysis and assessment of the state of the ocean are practically impossible without prognostic models controlled by Artificial Intelligence. Such models are trained on historical data, sensors, and satellite observations and combine complex data sets. They can predict the likelihood of environmental disturbances, areas of plastic pollution, or sea heat.
The use of recurrent neural networks (RNN) played a significant role in preventing negative changes in the ocean ecolandscape. Thanks to this, it was possible to respond in time to El Niño or coastal erosion. Models learn to process current and past conditions to create forecasts. This makes it possible to investigate the impact of terrestrial events on changes in the marine environment.
The integration of Artificial Intelligence with sensor technologies, satellite imagery, and predictive modeling has the potential to revolutionize this field. Researchers and scientists can get enough verified information to respond in time to the challenges in the marine environment and take care of its preservation.
Impact AI on Marine Industry
Earlier in our articles, we already raised the topic of the impact of Artificial Intelligence and the Shipping Industry. Read our previous articles “Benefits of Machine Learning in Port Operations” and “Data Science in Maritime Industry: Restructuring of Processes” and share your impressions.
Amazinum Case of Harnessing AI and ML in the Maritime Industry
About the Client
The company, to which Amazinum provided its data scientists, is engaged in the optimization of processes in shipping. It is the developer of certain pro-game solutions, services and platforms that have changed the policy in the maritime market.
Business Context
Data Scientists joined our client’s team to implement Data Science and Data Analytics solutions. Clients set our specialists the task of structuring data on ship traffic, information on berths and ports. Specialists developed a corresponding solution that simplified and automated the work of users in the shipping industry.
Our customers set us the following tasks:
- Predict the price of unloading and loading goods onto the ship;
- Determine the reliability of ports;
- Sorting varieties by similarity;
- Predict patterns and popularity of ports.
Predict the Price of Unloading and Loading Goods Onto the Ship
The Amazinum Data Scientists team was already working with ready-made data provided by the client. The task set before us by the customer was to predict unloading or loading prices based on certain metrics. To fulfill the tasks, our specialists took data on port services for the previous time and trained models based on them. Among the selected models were the Prophet model, which predicts time data based on seasonality, and the Darts library for time-series forecasting and anomaly detection using state-of-the-art models.
Among the metrics that Amazinum specialists took into account were:


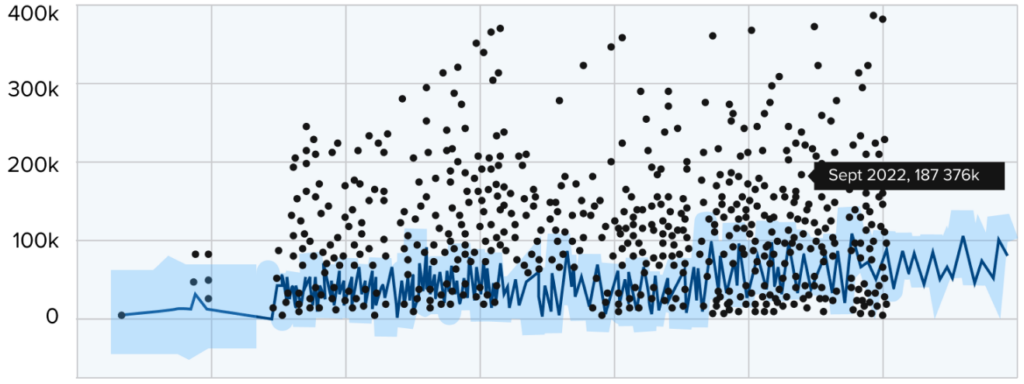
Date The scientists checked the results and provided the data to the client in the form of graphs.
Determining the Reliability of Ports
The next task that the client set before us was to determine the reliability of the ports. For this, Amazinum’s Data Scientists collected statistics on price fluctuations, such as mean value, standard deviation and variance.
Ports were ranked by these values and reliability was determined using certain metrics. Such indicators were the deviation per port and the standard deviation for each country, continent, etc. Based on them, the risk level of the ports was determined.



Companies that Use Artificial Intelligence For the Purposes of Developing Oceanography and Cleaning the Oceans
MBARI and Ocean Vision AI
In 2022, the MBARI Research Institute received funds for a new program called Ocean Vision AI. It uses Artificial Intelligence and Machine Learning to speed up the processing and access of ocean videos and images. This should help make maritime management more efficient.
To create a machine learning platform to help process underwater visual data, Ocean Vision AI combined its expertise with MBARI, the Northern and Central California Ocean Observing System (CeNCOOS), Climate Change AI, CVision AI, the Ocean Discovery League, and the University of California at Santa Cruz.
Kakani Katia, Principal Engineer at MBARI and Lead Principal Investigator of Ocean Vision AI, says of this collaboration: “MBARI and our collaborators are excited to harness the power of artificial intelligence, social science and games to accelerate the analysis of ocean data. Together, we are developing the tools that are urgently needed to help us better understand and protect our blue planet.”
In this case, Artificial Intelligence helps to solve the problem of a huge amount of data that needs to be analyzed. This is in particular data with complex scenes and organisms that require expert classification.
“Only a fraction of the hundreds of thousands of hours of ocean videos and images have been fully reviewed and analyzed, let alone shared with the global scientific community,” said Kathy Croff Bell, founder and president of the Ocean Discovery League and co-principal investigator of Ocean Vision AI. “For one dive, thousands of species could be registered in new places, at new depths and in new ecological conditions. A wealth of untapped biodiversity information is contained in these existing records, with new data being collected every day.”
The program has the following functions:
- Provide research groups with underwater images of Artificial Intelligence and open data.
- Create data pipelines from existing image and video data repositories
- Creation of video games to engage the public
- Create data products that will be distributed to open data repositories.
In this way, eco-activists, scientists or researchers get access to verified annotations and trained algorithms through open source platforms such as FathomNet. This technology will help process ocean images in real-time mode, while expanding the horizons of observation with the help of AI and ML technologies.
Liquid Robotics
Liquid Robotics has created the Wave Glider, a long-term ocean robot. It helps scientists, businesses or governments gain new knowledge about the marine environment and make the right decisions. With their underwater sensors, these autonomous robots are expanding the way we collect ocean and marine data. The Wave Glider is currently the world’s most proven autonomous vehicle.
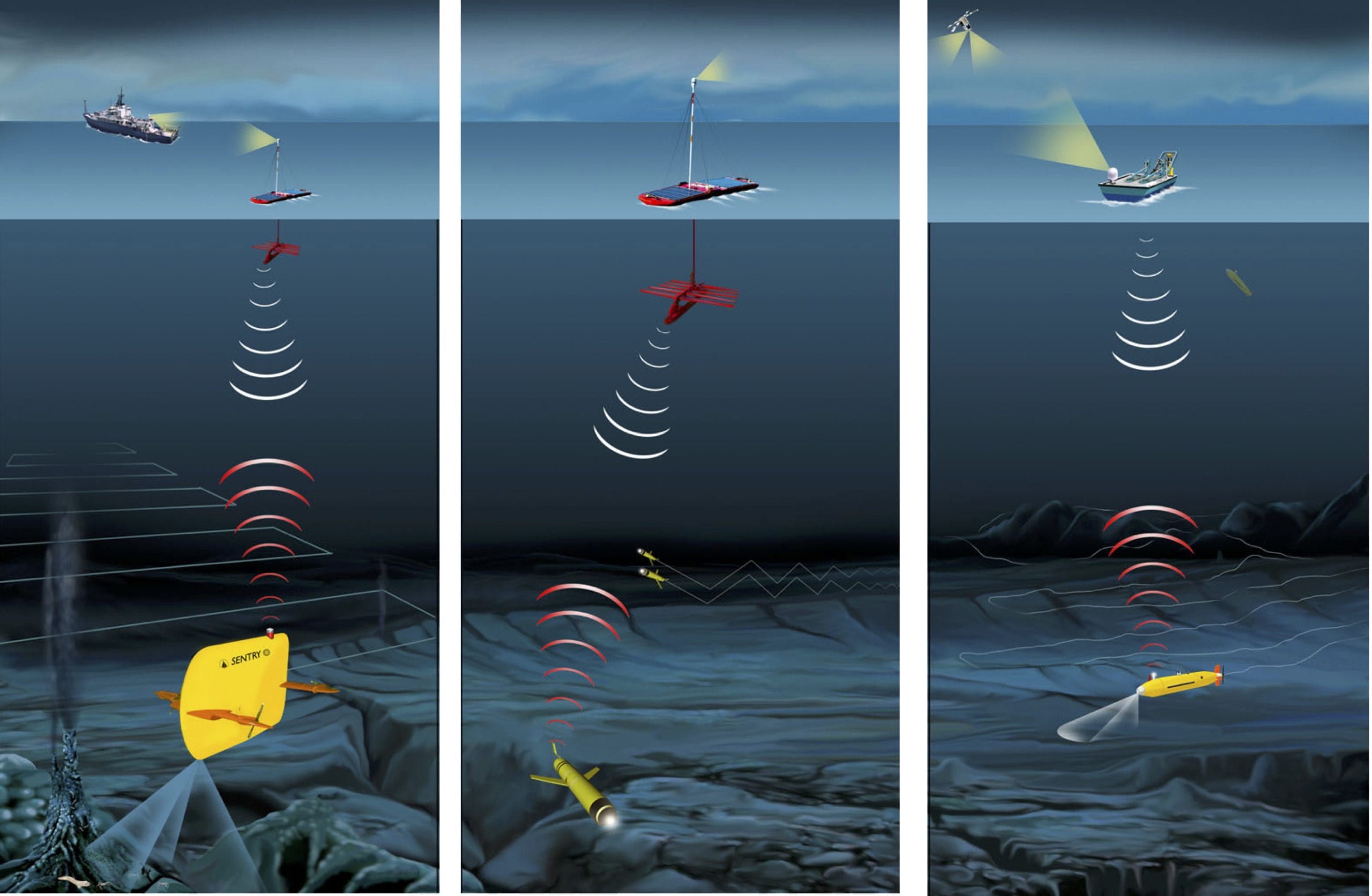
Source: Link
Currently, Wave Gliders, with sensors for monitoring and data collection, help industries:
- Defense – Anti-Submarine Warfare (ASW), intelligence, surveillance, and reconnaissance (ISR), and other military applications.
- Maritime Security – Maritime border protection (e.g., dark target identification), illegal fishing, trafficking and smuggling support, and marine protected area (MPA) monitoring.
- Environmental Assessment – Metocean monitoring to support scientific and commercial projects for climate, weather, marine mammal tracking, and offshore energy operations.
How does it work?
The Wave Glider receives waypoints and mission plans using the WGMS web application provided by Liquid Robotics. The robot moves with the help of a float on the surface, which is somewhat similar to a surfboard, an underwater cover with spring blades and wave energy. Solar panels on its float power Linux-based computers as well as other communications tools. The Wave Glider is equipped with a GPS and weather station, as well as a set of communication antennas. This is necessary to determine its exact location. In addition, it is able to automatically avoid ships, and capable of traveling long distances (2,500+ miles) and controlling large areas without refueling.
The Wave Glider is powered by a unique two-component architecture that uses the difference in wave energy at the surface of the water and at depth. It uses solar energy for added power and accuracy. Additional thrust increases mobility and accuracy and helps to navigate in difficult ocean conditions. Sensors and batteries are also powered by the solar energy system.
The Wave Glider is designed to support a variety of sensor payloads. In this way, the data is transmitted via satellite, and the continuous presence on the surface means that the data can be delivered as it is collected.
OceanMind
OceanMind is a non-profit organization that aims to reduce unregulated overfishing using satellites and Artificial Intelligence. They work with governments and local authorities to detect wrongdoing in the seas. In 2019, OceanMind joined the Microsoft AI for Earth program. This gave them the opportunity to discover new resources and solutions for their purpose.
A partnership with Microsoft has enabled OceanMind to process real-time vessel tracking data around the world. This allows them to report inappropriate fishing activities to local authorities and provide guidance.
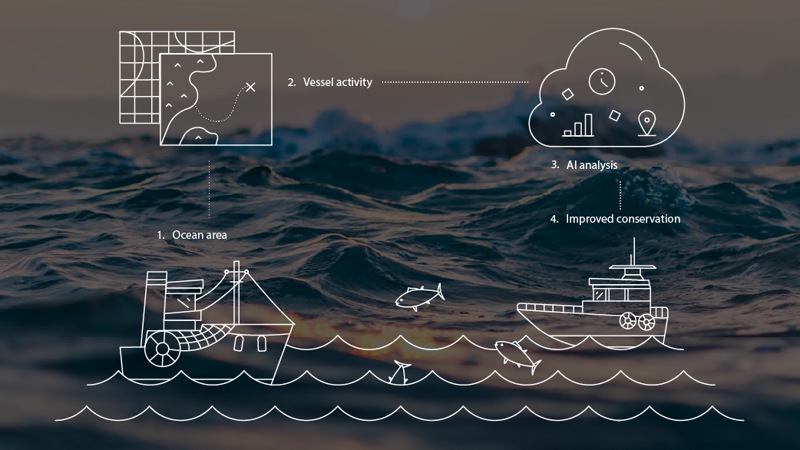
OceanMind uses Microsoft Azure cloud solutions to search for ships around the world that have been in contact over some time. Vessels are required by law to have AIS for safety reasons. The ship’s identification code determines its destination. It is transmitted by the AIS signal. In this way, technologies process geospatial data from the automatic identification system (AIS) of each vessel. In this way, OceanMind can identify rogue vessels that attempt to manipulate information.
How does it work?
A cargo ship moving at low speed or in a circle indicates suspicious behavior. The AI system and analytics detect and investigate anomalous behavior.
Sudan may turn off its surveillance systems. In this case, OceanMind uses satellite imagery, namely Synthetic Aperture Radar (SAR) technology. SAR uses radio waves to create three-dimensional reconstructions of objects. The echo of each pulse is received and recorded through a possible target scene. The antenna position changes relative to the target as the SAR device moves with the spacecraft. All this together allows you to create multidimensional images of ships with high resolution.
OceanMind uses a special form of R-Trees to store data. Its essence consists in grouping neighboring objects and representing them with a minimal bounding rectangle. OceanMind uses R-Tree with Update Memos (RUM-tree) for continuous updates.
When two or more vessels are in close proximity, a proximity warning is generated. ML algorithms then detect illegal activities. Using artificial intelligence that analyzes billions of data points, OceanMind obtains information about non-compliant vessels that may constitute potential illegal fishing. It analyzes the exact time, the wrongdoing, the type of suspected fishing vessel and the exact violation.
Sofar Ocean
Sofar Ocean is a marine weather forecaster and has deployed an array of 200 weather sensors in the Pacific Ocean to provide the most accurate wave forecast in the world.
Company operates the world’s largest private network of ocean weather sensors. There are about 200 of them, and all of them are designed to improve ocean information and forecasts. Each of the sensors must measure wave conditions, surface wind, and drift currents in real-time and transmit the data to the Sofar cloud. All real-time sensor data is available at weather.sofarocean.com
Compared to the NOAA models, the Sofar data network reduces model RMS errors by more than 20% in all directions and by more than 50% in high-energy swell forecasts. The best part? All of our real-time sensor data and advanced model forecasts are publicly available at weather.sofarocean.com
The Sofar team is working to reduce the ocean data gap. For this, they created a modern API for seamless integration into simulation systems and real-time access around the world. They continue to collaborate with academic research groups to provide ocean data, improve model predictions, and provide vast amounts of ocean data.
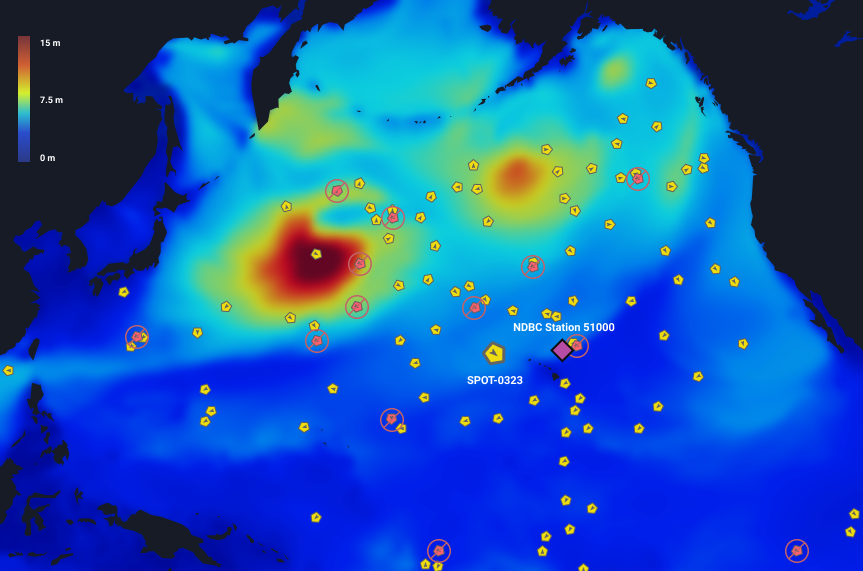
Source: Link
How It Works?
Predicting the size and time of arrival of waves is an important aspect of coastal defense and the safety of maritime operations. National Oceanographic and Atmospheric Administration (NOAA) forecast centers use statistical wave models (WaveWatchIII) for this. However, the predictions from these sensors are often inaccurate, they do not correctly determine the height of the wave or the time of its arrival. To compensate for this, Sofar pulls all available data from its network into its version of a global wave forecast model. The data-driven model has significantly less error and performs better than NOAA. At the same time, the difference between NOAA and Sofar is only in the data.
In 2020, the Sofar team deployed 200 Spotter sensors in the Pacific Ocean basin. They collected real-time data on waves, wind, surface drift, and water temperature (SST). This kind of sensor network is currently unique and represents the largest and most advanced private ocean data network in the world.
In addition, Sofar has set up its own global numerical weather models. For example, for ocean waves, their team runs the same model as NOAA (WaveWatchIII + ST4 physics), while feeding their sensor data into the model in real-time. The process of adding data is called assimilation.
Sofar explains: “To keep things simple, we started by using a widely used technique called optimized interpolation (OI). Essentially, every time we run a forecast cycle, we adjust the initial conditions (the current state) to better match the observations in regions where we have sensors.In this way, we “push” the model to follow the measurements as closely as possible. This information is then propagated forward in time (forecast) using a numerical physics model. This is a very simple but effective method of integrating observations into model predictions.”
Orbital Marine Power
In 2021, the Scottish company Orbital Marine Power proposed an innovative design solution for tidal currents. It is the introduction of a 2MW O2 Orbital O2 tidal turbine, which has the potential to become the most powerful operating tidal turbine in the world. The turbine can generate net estimated electricity and power around 2,000 homes in the UK. Learn more about it here.
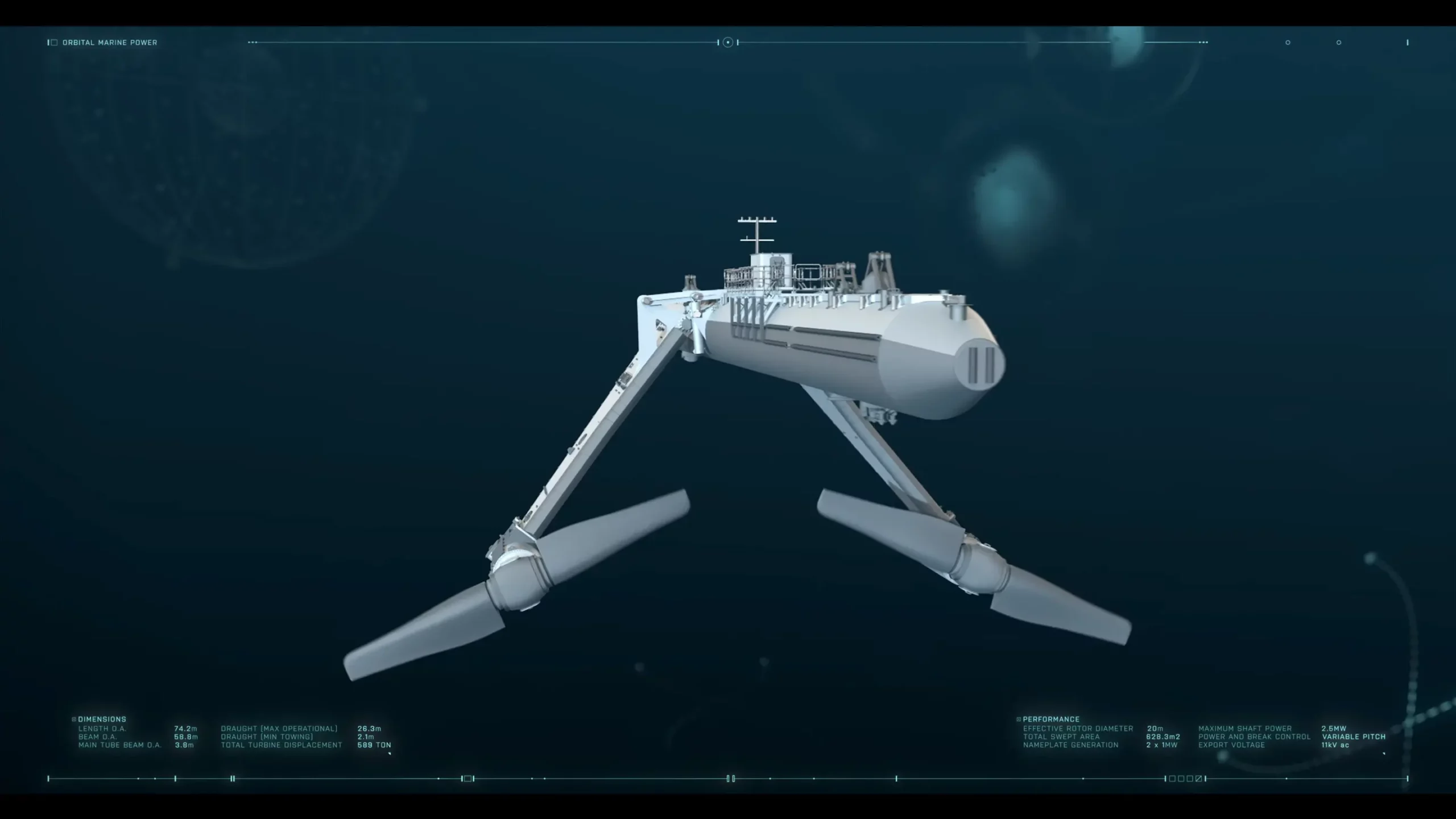
Source: Link
Andrew Scott, CEO of Orbital Marine Power, says: “Orbital is committed to ensuring that tidal energy makes a tangible contribution to the decarbonization of energy systems while supporting a just transition by creating a new industrial sector. The approval of this selection is a huge boost to this goal and allows us and our project partners to accelerate this positive vision. We look forward to EURO-TIDES developing at this rate in early 2024.”
Solar and lunar energy play a major role for Orbital. The Moon’s gravity pulls on the Earth’s surface, lifting vast amounts of ocean water around the globe in predictable patterns. Passing through narrow gaps or around capes, the water accelerates. This allows kinetic energy to be collected using turbines under the surface of the ocean. This has a name – tidal force.
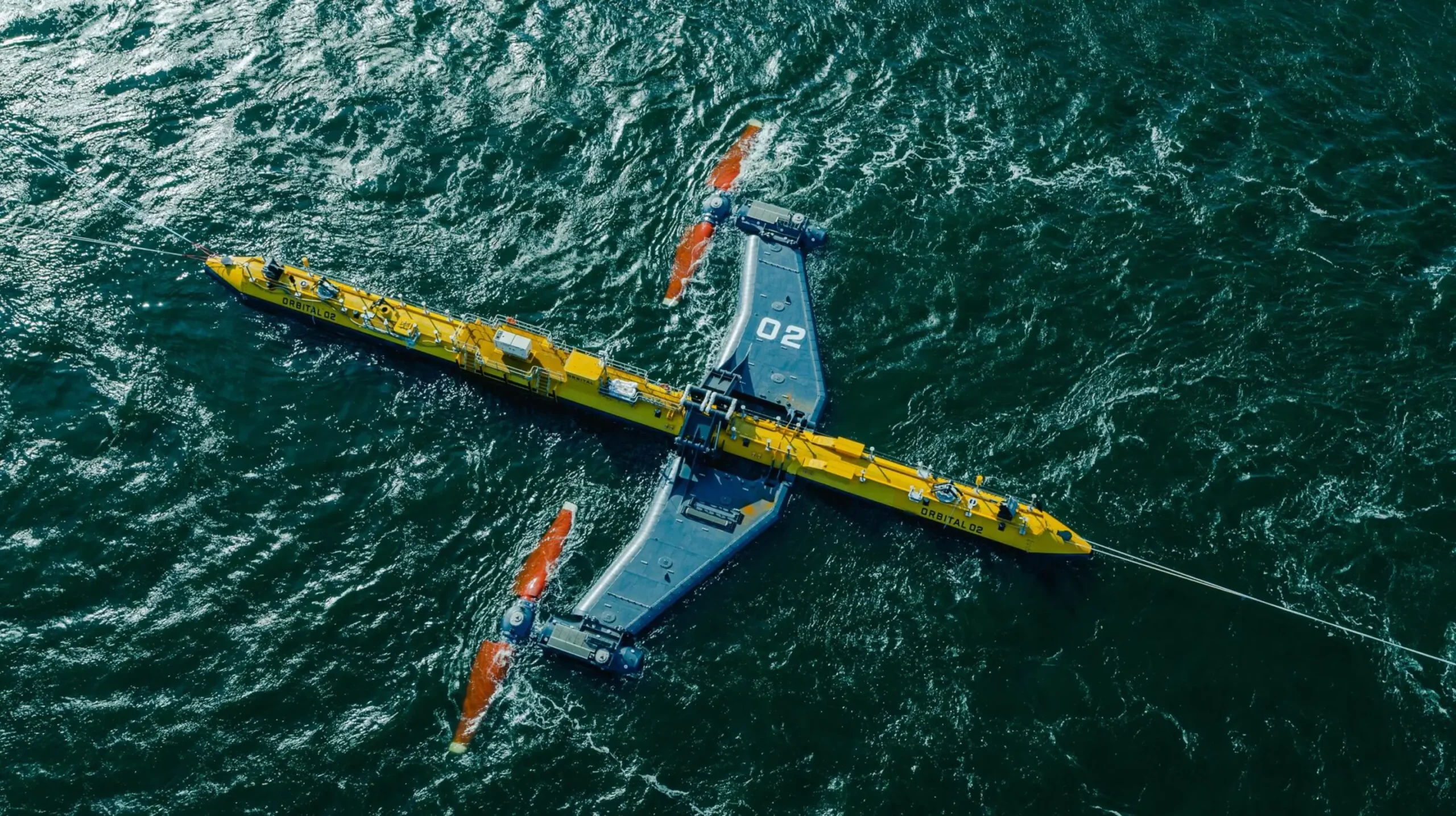
Source: Link
Orbital aims to reduce costs as much as possible. Their ships have floating turbines that are located in the canals and accelerate the tidal currents. The blades, connected to the main platform, which in turn is connected to a turbine, can change the tides and generate electricity. Underwater cables carry it to the shore.
The company says: “We can generate enough clean, predictable electricity to meet the demand of around 2,000 UK homes and offset around 2,200 tonnes of CO2 production per year.”
Why Impact AI on Marine Ecology is important?
There are several important reasons why the application of AI technologies is crucial for ocean safety, cleanliness, and research.
Data Analysis and Prediction
Artificial intelligence (AI) algorithms are able to efficiently analyze large volumes of oceanographic data. They receive data from a variety of sources, including satellites, research vessels and autonomous cars.Artificial intelligence (AI) can process this data and offer insightful information about marine ecosystems, climatic patterns, and ocean dynamics. Researchers can anticipate changes in ocean conditions, such as sea level rise, storm surges, and harmful algal blooms, with the use of AI-enabled predictive analytics. This can help with proactive decision-making and risk mitigation strategies.
Environmental Monitoring and Management
By examining variables including temperature, salinity, biodiversity, and water quality, AI-powered monitoring systems can continuously evaluate the condition of marine environments. Early detection of environmental threats such as pollution, oil spills, and illegal fishing is made possible by real-time monitoring. AI protects marine biodiversity, restores and preserves marine ecosystems, and supports sustainable fisheries management by quickly recognizing and mitigating these threats.
Safety and Navigation
By enhancing navigational systems and reducing operational risks, artificial intelligence (AI) technologies improve safety for maritime activities. Algorithms driven by artificial intelligence (AI) can examine weather predictions, vessel traffic patterns, and oceanographic data to enhance route planning, steer clear of dangerous situations, and avert maritime collisions. AI-based navigation systems enable autonomous boats to operate more effectively and safely, lowering the risk of mishaps and boosting maritime security.
Reaction to Environmental Disasters
Artificial intelligence (AI) technologies are essential for organizing emergency response activities and reducing environmental damage in the event of environmental disasters like oil spills, hurricanes, or marine accidents. Artificial intelligence (AI)-enabled modeling and simulation tools can forecast the impact of pollutants on marine ecosystems, model the spread of pollutants, and optimize response plans for containment and cleanup efforts. Emergency responders can more efficiently coordinate resources, lessen their impact on the environment, and speed up the recovery process by utilizing AI-driven decision support systems.
Policy Making and Resource Management
With the use of AI-powered analytical tools, decision-makers in these fields can make well-informed choices based on thorough data analysis and scenario modeling. Policymakers can develop evidence-based regulations and conservation measures to promote ocean sustainability by evaluating the cumulative impacts of human activities and comprehending the intricate interactions within marine ecosystems. AI-based predictive models facilitate long-term planning for sustainable ocean use. They strike a balance between environmental conservation goals and economic development.
Conclusion
One of the most remarkable outcomes of artificial intelligence in oceanography is the notable increase in the capacity to investigate and comprehend the world’s oceans. Through the use of AI-enhanced autonomous underwater vehicles (AUVs), the deep sea—a region once shrouded in mystery due to logistical and physical barriers to exploration—is becoming more accessible.
Geographically speaking, AI has democratized oceanographic research by enabling even smaller research institutions to access high-throughput data analysis. This has made oceanography more globally collaborative, allowing different research groups to share and benefit from a common pool of ideas and data.
Artificial Intelligence is able to process huge volumes of multidimensional data and, thanks to this, develop complex integrative models of oceanic systems. Artificial intelligence’s predictive analytical powers go beyond providing assessments of the present to include forecasting future ocean conditions. These predictive models are essential for organizing and overseeing actions meant to lessen the effects of climate change on marine environments.