Ever-increasing volumes of data today plays a decisive role for every company that wants to stay in top. That means employing artificial intelligence, machine learning technologies in data science, to prepare and organize big data more effectively and rationally.
To do this efficiently and rationally, specialists and analysts use additional tools that provide an advantage in one way or another: speed of data processing, comfortable work with many sources or other. Learn more about the history of five tools that have grown from a startup level to a successful project today.
Arrikto
Arrikto was founded in 2014 and already in 2020 has raised $10 million in series A funding.
Unlike other MLOps companies, Arrikto allows accelerating machine learning models to market 30-times faster than traditional ML platforms. As a leading Kubeflow contributor, it provides automated workflows, reproducible pipelines, consistent deployment from desktop to cloud, and secure access to data. Arrikto’s Enterprise Kubeflow is available as a multi-node distribution on AWS, GCP, and Azure. It is the preferred MLOps platform used in production today by many companies.
Arrikto’s flagship product, Arrikto Enterprise Kubeflow, is a complete machine learning operations (MLOps) platform. One of the tasks is to bring together data scientists and DevOps to simplify, accelerate and secure model development through production. The goal, according to the company, is to bring the same principles used in DevOps to machine learning data. The company also offers the cloud-native Rok Data Management Platform to manage the data.

Comet.ml
Founded in 2017, today MLOps startup Comet announced that it raised $13 million in a series A funding round by Scale Venture Partners. The company plans to put toward product, sales, marketing, and engineering growth.
Comet develops a self-hosted, cloud-based MLOps platform for machine learning model development and monitoring. The company focuses on the three core elements of development: experiment management, model management, and production monitoring. The customizable ML development platform allows users to manage and optimize models across the entire ML lifecycle in a single user interface.
Comet offers a new ML development stack that helps businesses to grow and avoid many challenges. These tools are very useful for data scientists, engineers, and team leaders. They can track, compare, explain, optimize deep learning models or production models. Also they have an opportunity to manage related datasets. In addition, this tool allows users to share their work, iterate, and reproduce results so they can build better models faster.
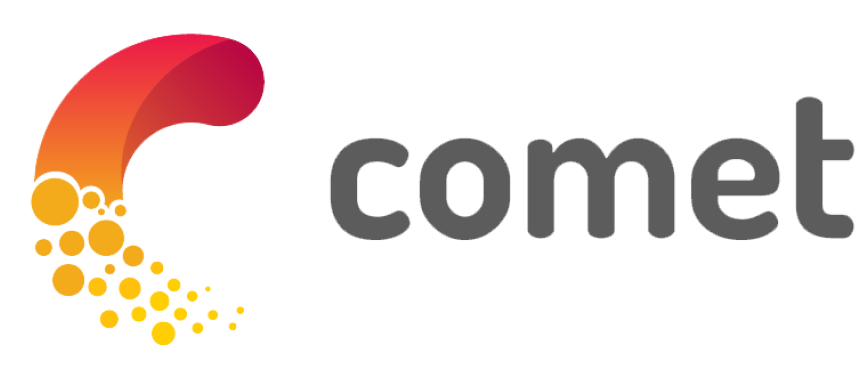
Databand.ai
Founded in 2018, Databand.ai raised $14.5 million in December 2020 in a series A round of funding led by Accel.
Databand’s unified data observability and machine learning development platform are helpful for data engineers and data scientists. Using these tools they have a wide field of action: identify, troubleshoot and fix data quality issues for data pipelines running on cloud-native systems such as Snowflake, Apache Spark and Apache Airflow. One of the main tasks is to help data engineers scale their infrastructure while maintaining data health standards so their organizations can build better data products.
Since Databand was acquired by IBM, Databand’s proactive data observability platform further extends IBM’s existing data fabric solution. It helps to ensure that the most accurate and trustworthy data is being put into the right hands at the right time – no matter where it resides.
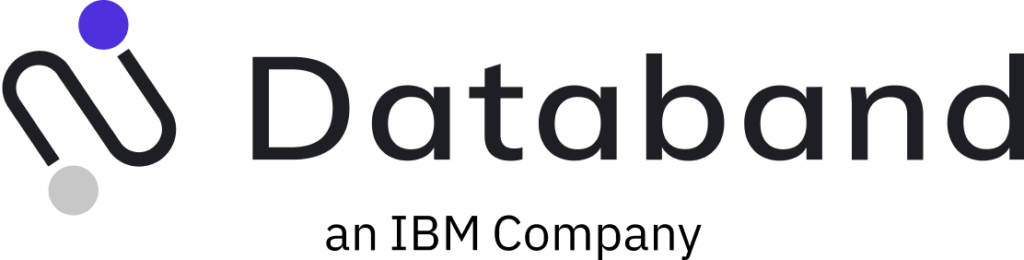
dotData
The dotData company was founded in 2018 as a spin-off of NEC Corporation.
At first was launched dotData Cloud, an AI/ML automation platform and services. The small organizations picked it up as something useful that helps to quickly automate artificial intelligence and machine learning development tasks. And in May the company debuted dotData Py Lite, a containerized AI automation system for data scientists using Python.
dotData develops what it calls AutoML 2.0 solutions for automating data science workflows. The dotData Enterprise machine learning and data science automation platform handles data ingestion and wrangling. Also it automated feature engineering, AutoML and model operationalization tasks. The main advantage – all with zero coding. DotData delivers end-to-end data science automation for the enterprise. Its data science automation platform speeds time to value. It works thanks to automation of the entire data science process.

Explorium
Explorium, founded in 2017, its total financing now is $127 million.
It develops an automated external data platform for advanced analytics and machine learning tasks. The system enriches the data you have by introducing you to data sources that boost your predictive models’ accuracy. It automatically integrates thousands of relevant external, partner or public data sources. Accordingly you can build superior, more accurate predictive data models through better data. Also it provides all the details that you need to decide whether to use this data feature set with your own model or with one of the models generated by Explorium.
The company automates the process of data wrangling, collects the data from various databases, joins it, orders it, sorts it, normalizes it and applies Explorium’s proprietary Computer Science ontology. The feature recommendation engine tests hundreds of thousands of potential data features and identifies those that contribute the most value to accurate predictive results.
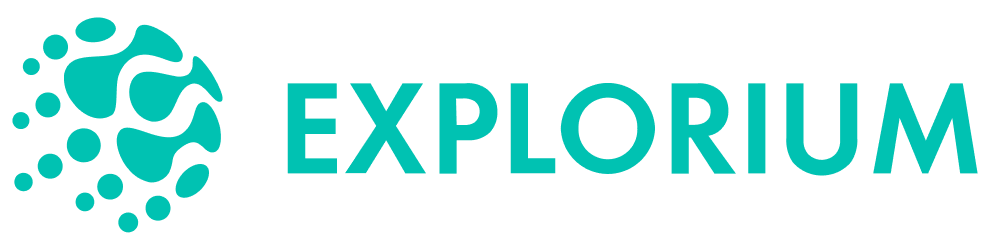
Conclusion
Additional tools greatly simplify tasks that involve a large amount of data. It also saves a lot of time and increases efficiency. Thanks to the development of such tools, the industry is moving forward much faster.
You have already seen 5 tools that were small projects early on. And no one even thought that they would grow so much and blow up the industry. Tell us about your startup and the Amazinum team will help you realize it.