Recommender System
Build better interactions with your customers by analyzing past likes and dislikes, product interactions and behaviors, and more. Amazinum will help you with this.
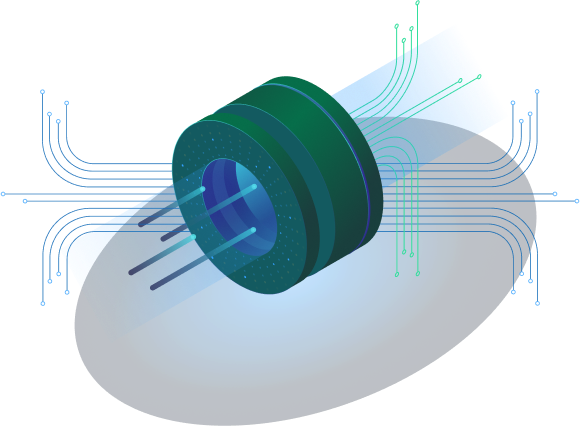
Recommender systems are subclasses of information filtering systems that can offer you any content related to your user experience. Ranked item lists are just the simplest form of personalized recommendation. For this, recommender systems try to provide the user with the most suitable products based on preferences and user experience.
Recommender systems are built on user information, product information, and transaction information (or any similar actions). RS can use different algorithms, including collaborative filtering, content-based filtering, and hybrid systems that combine both methods.
The main goal of recommender systems is to personalize the user experience by providing highly relevant and useful recommendations. However, they can also be used for:
- Aids in decision-making
- Aids in comparison
- Help in opening
- Intelligence assistance
- Calculation of behavioral aspects of the user
Thus, the recommender system is an important component of business. The Amazinum team can help you increase revenue and profitability, or optimize your product portfolio.
We are Experienced at
Amazinum specialists will help you implement any model of Recommendation systems according to the requirements of your business. There are 3 known teams of recommender systems.
Collaborative Filtering
A technique that uses the preferences of other users to make recommendations to a specific person. The idea is that people who have shared preferences in the past are likely to share them in the future. The collaborative filtering method is based on the collection and analysis of user behavior data.
There are two types of collaborative filtering methods:
- User-based collaborative filtering – an algorithm that provides recommendations based on users with the same preferences as the target customer.
- Item-based collaborative filtering – an algorithm that recommends items similar to those that the target user has already liked.
Thanks to the vast amount of data about how users interact with a certain item, collaborative filtering algorithms can identify similar users and provide recommendations. Moreover, they use similarity scores between users to correctly predict the interest of the target user.
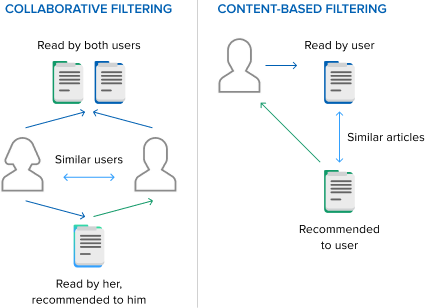
Content-Based Filtering
Content-based filtering is a filtering method based on product content and user selection. That is, the characteristics of the elements that the user liked in the past are key for this method. This approach can be applied to any type of element that has explicit or implicit attributes. The system uses keywords to describe the product, and a user profile is created to reflect the user’s preferences. The basic thesis and assumption of this method is that if you like a certain item, you will like a similar item.
Algorithms in this method use previous user behavior, such as ratings, likes, or purchase history, to create a profile of user preferences. This profile becomes the basis for recommendation items that have similar attributes to those that have been liked by the user.
Hybrid Recommendation Systems
Hybrid systems use several methods at the same time – content-based filtering and collaborative filtering to provide more accurate and diverse recommendations. A more personalized and personalized experience makes the hybrid method the most promising and accurate. hybrid information systems may incorporate demographic data, contextual data, or external data sources to provide more accurate recommendations.
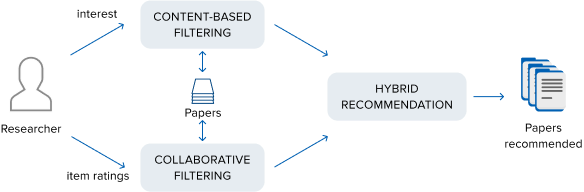
Business Value
The Amazainum team will provide you with qualified specialists who will help you improve your interaction with your customers, improve your services, and increase your income. By implementing the recommended systems in your business, you will receive several benefits.
- Personalization
Users will receive more personalized and unique recommendations based on the user’s past behavior, preferences, and interactions. This will allow you to be more sensitive to the user’s needs and satisfy his personal wishes. - Increased interaction
By providing a user with content, products, or services that they will be interested in, recommendation systems can help you increase engagement with your users, maintain engagement, and increase sales or conversions. - Improved user interaction
Facilitate your user’s experience by recommending the content, products, or services they are looking for. This will increase user satisfaction and win their loyalty to you. - Increase in income
Personalized recommendations will help your business increase revenue, sales, and conversions. You will increase the chances of a sale, attract the attention of users, and gain their trust. - Better understanding of user behavior
Understand your user’s behavior and easily adjust your marketing strategy, product development, business decisions, and resources.
Recommender Systems: Use Cases
E-commerce
The system will help provide special offers to users based on their purchases, as well as recommendations based on users with similar tastes.
Entertainment
Recommendation models are used by entertainment services to recommend content that may be of interest to the user. By analyzing consumer behavior, provide offers that will suit your user’s needs.
Social media and news
News platforms use recommendation systems to recommend articles, videos, and other content that will be of interest to the user. In addition, RS can also be used in social networks to provide the user with recommendations of friends, groups, or publications.
Healthcare
With recommendation systems, you can provide personalized and accurate recommendations for treatments, prescriptions, and other medical services.
Fintech
You can provide advice on investments, credit products, and other important financial aspects.
Advertising
You can provide personalized recommendations and offers that will interest your user. In addition, you can better select your target audience and adjust your advertising to a specific user segment.
Companies that Already use Recommender Systems
The flexibility and price of cloud platforms have led to the widespread popularity of their use and implementation in their services. Here are a few companies that have implemented cloud-based solutions into their services.
Netflix
Another data-driven business that uses recommendation algorithms to increase consumer satisfaction is Netflix. According to the same Mckinsey study that we just discussed, recommendations account for 75% of Netflix viewing. Netflix is so focused on giving its customers the greatest experience possible that it has organized data science competitions dubbed Netflix Prizes, where the winner of the most accurate movie recommendation algorithm receives a prize money of one million dollars.
Spotify
Each week, Spotify creates a fresh, personalized playlist called “Discover Weekly” for each subscription. This playlist consists of 30 songs chosen specifically for each user based on their individual musical preferences. Through their acquisition of the music intelligence and data analytics business Echo Nest, they were able to develop a recommendation engine for music that employs three distinct recommendation models:
- Collaborative filtering is the process of selecting songs based on a comparison between individual listeners’ past data and that of other users.
- Natural language processing: gathering data about particular musicians and songs from the internet. Then, a dynamic list of the most popular terms is assigned to each artist or song, which is weighted by relevancy and is updated every day. Next, the engine decides if two musical compositions or performers are comparable.
- Audio file analysis: The system analyzes the time signature, key, tempo, and loudness of each unique audio file and provides recommendations based on those findings.
Hulu
Based on user ratings, viewing history, and preferences, Hulu uses recommendation algorithms to offer TV series and movies to users.
Goodreads
Using recommendation algorithms, Goodreads makes book recommendations to users based on their reading preferences, reviews, and ratings.
Fitbit
Fitbit uses recommendation algorithms to provide users with tailored exercise regimens, health advice, and fitness goals based on their activity levels, goals, and health information.
LinkedIn makes recommendations in the form of “You may also know” or “You may also like,” just like any other social media platform.
Amazon
The majority of the pages on their website and email campaigns on Amazon use item-to-item collaborative filtering recommendations. 35% of Amazon purchases, according to McKinsey, are made possible by recommendation algorithms.
Waze
Based on user reports, historical data, and location, Waze is a navigation app that employs recommendation systems to offer users real-time traffic information and recommended routes.
FAQ
Are recommendation systems an example of AI?
A recommendation system is a machine learning-related artificial intelligence (AI) algorithm that utilizes big data to suggest or recommend other products to customers. These can be determined by a number of criteria, such as previous purchases, search history, demographic data, and other elements.
How do recommender systems work?
By collecting data about people’s interactions with products, recommender systems are trained to understand people’s preferences, past choices, and product attributes. These consist of buys, likes, clicks, and impressions.
Who uses recommender systems?
There are numerous applications for recommendation systems in a variety of industries, such as e-commerce In order to offer customers personalized product recommendations based on their past behaviors and preferences, recommendation systems are widely used in eCommerce.
Amazinum Portfolio
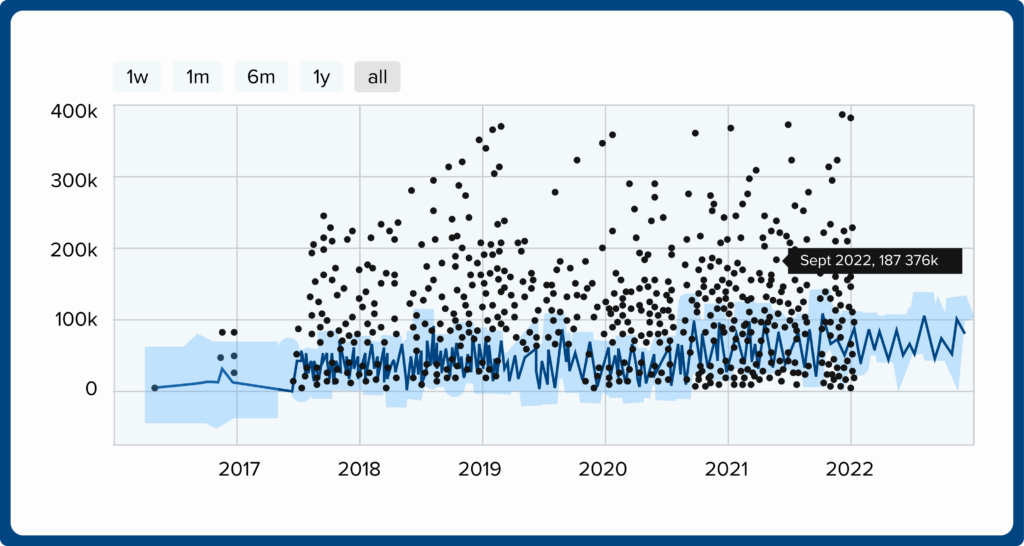
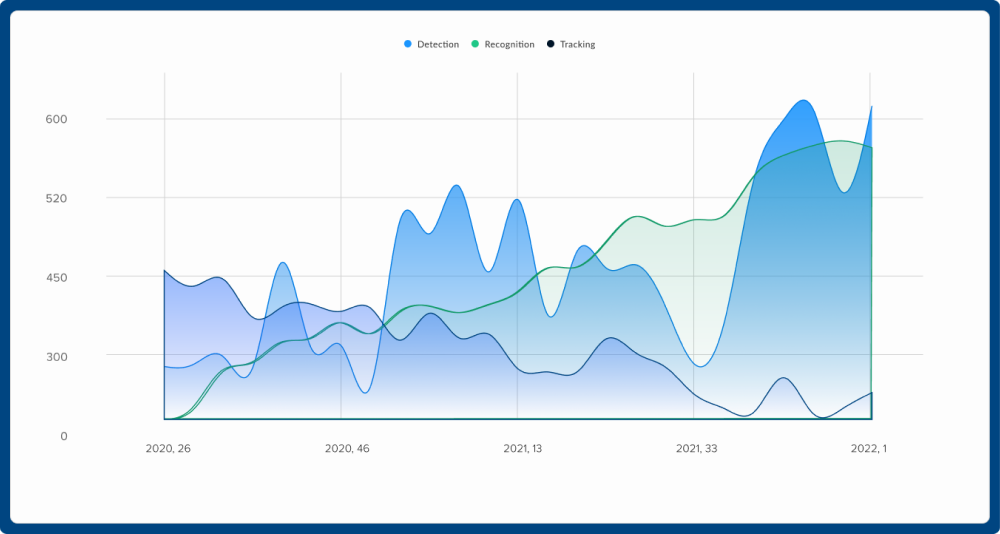
Smart Eyes: The Integration of AI and ML in Surveillance Cameras for Enhanced Security
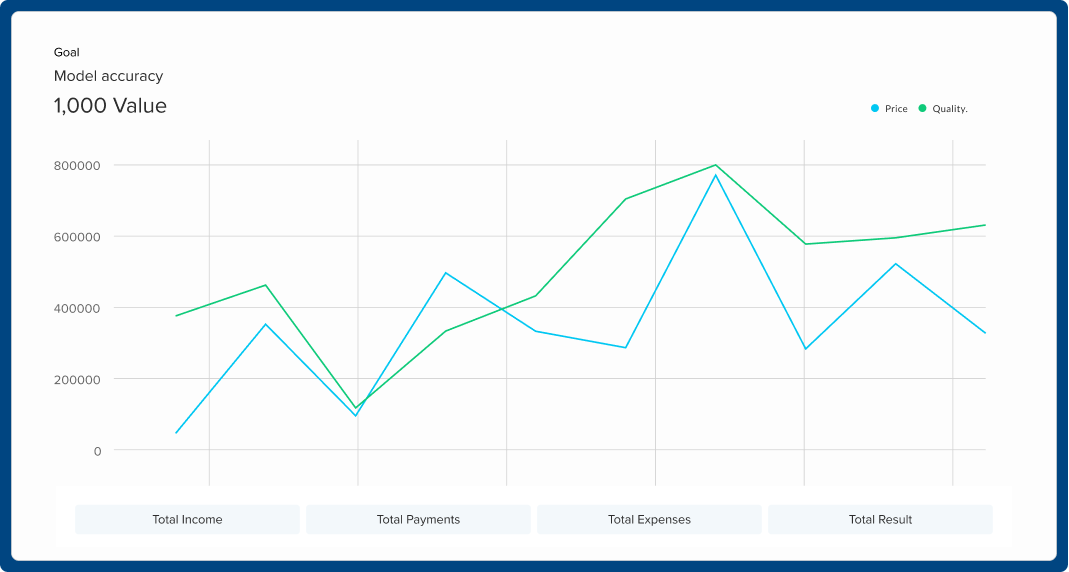
Beyond Monitoring: The Power of AI and ML in Construction Site Surveillance
Our Industry Focus
Our industry knowledge and background give our clients and partners confidence that we understand their business. Here we highlighted a few top industries we are good at, penetrating to the smallest details and nuances of a certain branch.
SEO & Marketing
Healthcare
Safety & Security
Sport
E-commerce & Retail
Gambling and Casino
Manufacture
Ecology
FinTech
Energy
Localisation
IoT
Partners & Clients
We deeply appreciate our partners for cooperation. Every member of the Amazinum Team does their best to provide the highest quality of services and solutions. We satisfy all your needs and requirements.
Technologies
Learn about technology stack we use to implement data science:
Programming languages:
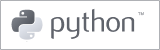
for data analysis and processing:
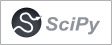
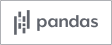
for creating solutions:


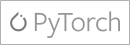
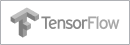
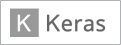

for API development:
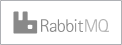
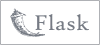
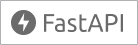

for visualization:
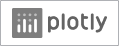
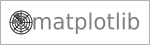
Deploying solutions:
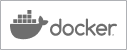
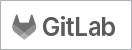
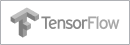

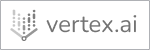

Databases:
SQL:
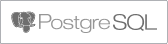
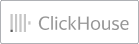


NoSQL:


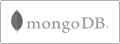

Cloud Solutions:


Vitaliy Fedorovych
CEO, Data Scientist at Amazinum
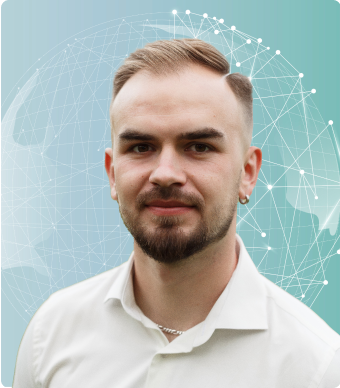
Hello there!
Amazinum Team assists you through all data science development processes:
from data collection to valuable insights generation.
Get in touch with our CEO and Data Scientist to figure out the next move together
- 4A Peremohy square, Ternopil, Ukraine, 46000
- +380 98 85 86 330
- vfedorovych@amazinum.com
Contact Us
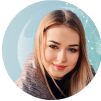
Bazarnitska Alina
Client Partner
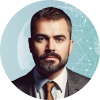
Ihor Khreptyk
Client Partner
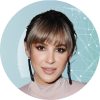
Stopnyk Zoriana
Client Partner
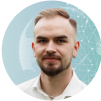
Vitaliy Fedorovych
CEO, Data Scientist